Unlocking the secrets of our genes: Best practices in genome-wide association studies
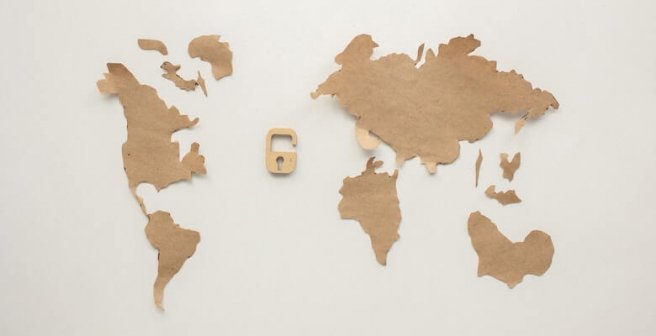
The human genome is like a blueprint that holds the key to understanding our health and well-being, so it’s not surprising that there’s been an explosion of different research methods combining genetics and statistics. Genome-Wide Association Studies (GWAS) are a powerful tool in deciphering the complex relationship between our genes and various traits, from disease susceptibility to personality characteristics. In this blogpost, we’ll walk you through some best practices in data analysis for GWAS, ensuring that you can embark on your genetic discovery journey with confidence.
Understanding GWAS
Before diving into data analysis, let’s grasp the basics. GWAS is a scientific technique that scans the entire genome, examining millions of genetic variants to identify associations with specific traits or diseases. These genetic variants, known as Single Nucleotide Polymorphisms (SNPs), can give us valuable insights into the genetic basis of a particular trait.
Quality Matters: Data Preprocessing
Your journey into GWAS analysis starts with data preprocessing. This crucial step involves cleaning and preparing your data to ensure accurate results. Here are a few essential tasks:
Quality Control (QC): Begin by checking for errors, missing data, and outliers. Remove low-quality SNPs and samples to avoid skewing your results.
Population Stratification: Our diverse world means genetic variations can differ between populations. It’s essential to account for this in your analysis, using methods like Principal Component Analysis (PCA) to correct for population stratification.
Statistical Power and Sample Size
In GWAS, size matters, and we’re not talking about your lab coat! To detect meaningful associations, you need a sufficiently large sample size. The statistical power of your study generally increases with more samples, making it easier to spot genuine signals among the genetic noise. However, note that recent research by Wang and Xu (2019) shows that depending on your research question, you may not need a very large sample for GWAS, provided you perform your power analysis strategically. Either way, power analysis is an important part of GWAS to avoid wasting time and resources on underpowered or overpowered research.
Choosing the Right Statistical Model
Now that your data is clean and you’ve got a sizable sample, it’s time to choose a statistical model. A commonly used model is logistic regression, which assesses the relationship between genetic variants and binary traits (like disease status). For continuous traits (e.g., height or weight), linear regression is often used. Linear mixed effect models are powerful tools used to consider how different groups of people might affect our results and to understand the genetic architecture of complex traits like disease susceptibility. For a comprehensive overview of different statistical methods used in GWAS, you can refer to Sun and Zhao (2020).
Multiple Testing Correction
Imagine flipping a coin a hundred times; you’re likely to get some heads purely by chance. Similarly, when you test millions of SNPs, some may appear significant by sheer luck. To combat this, you can apply multiple testing correction methods, such as the Bonferroni correction or False Discovery Rate (FDR) control, to reduce false positives. Various researchers have also developed sophisticated techniques to correct for multiple testing specifically in GWAS, such as Joo et al. (2016), Gao (2011), and Wei et al. (2009).
Data Visualization: Bringing Genes to Life
Numbers can be overwhelming, so don’t forget to visualize your findings. You can create Manhattan and Quantile-Quantile (QQ) plots to identify significant SNPs and assess overall data quality. Visualization can help you and others understand your results better.
Replication and Validation
Congratulations! You’ve found some exciting associations. Now, it’s time to replicate your findings in independent datasets. This step ensures your discoveries aren’t a one-time fluke and adds credibility to your study.
Interpreting Biological or Clinical Significance
Numbers are only half the story. You need to talk about the real-world implications of your associations. What do these genetic variants mean in terms of biology or clinical practice? Collaborate with domain experts or clinicians to shed light on the functional relevance of your findings.
Conclusion
Statistical rigor is essential in GWAS because it unveils links between genes and traits, informing medical research and personalized healthcare. It helps identify disease causes and develop targeted therapies, offering insights that can improve human well-being. By following these best practices, you’ll be equipped to uncover the secrets hidden within our genes, contributing to a better understanding of human health and genetics.
You can now unlock secrets in the human genome through personalized advice from a trusty biostatistician! Check out Editage’s Statistical Analysis & Review Services.