4 Statistical errors researchers should avoid at all costs
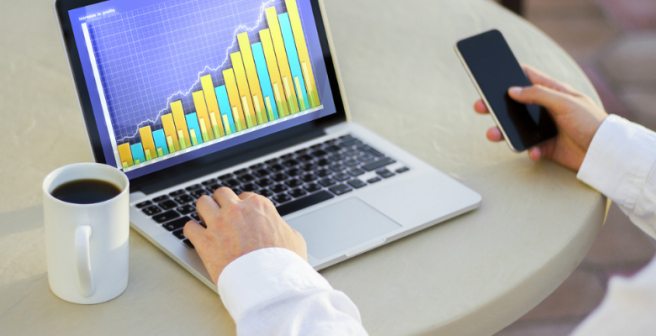
Statistics is a tool used for assessing relationships between two or more variables and evaluating study questions. Going a little deeper, biostatistics, a combination of statistics, probability, mathematics, and computing, is used to resolve problems in biomedical sciences. Application of biostatistics in a study enables researchers to analyze whether a new drug is effective, what the causal factors of the disease are, the life expectancy of an individual with illness, the mortality and morbidity rate in a population, etc.
Although statistics is one of the primary tools of biomedical research, its misuse and abuse, whether intentional or unintentional, is widespread. It is, in fact, increasingly being identified as one of the chief factors for manuscript rejection.
This article looks closely at the reasons behind misuse of statistics and ways to fix this widespread problem in biomedical research. Let us begin by understanding what lies behind statistical errors.
1. Lack of clarity in presenting statistical data: Manuscripts present statistical methods and analyzed data. However, there is a huge grey area that can prevent readers from getting a complete picture of the statistical data: many papers fail to state the statistical assumptions with clarity. In a cross-sectional study involving students and faculty of medical colleges, 53.87% found statistics to be very difficult, 52.9% could not correctly define the meaning of P value, 36.45% ill-defined standard deviation, and 50.97% failed to correctly calculate sample size. This indicates that it is important for researchers to not only analyze their data correctly but also to use and present it correctly.
2. Skewed emphasis in data vs. theory: Although clinical studies undergo rigorous peer review in terms of statistics, the same is not true for basic science studies. The interdisciplinary nature of basic research, which often involves biochemistry, behavioral science, animal models, as well as cell culture, makes statistical analysis challenging. Often, researchers decide on the use of statistical analysis only after performing their experiment. This strategy is similar to a post-mortem analysis and provides limited insights.
3. Poor decision-making prior to data collection: It is crucial to plan statistical analysis at critical stages of an experiment, for example, when deciding the sample size (e.g., number of mice). This can have significant implications on the outcomes of the study. Considering that there are several variables (e.g., weight, BMI) that can affect the outcome based on the sample, an efficient approach is to perform sample size computations for each outcome and then decide on the largest practical sample size. Investigators should ideally decide the analysis of the exposure-outcome relationship prior to data collection. This is an efficient way of avoiding false-positive relationships. Investigators should specify a primary outcome variable and decide whether their study involves comparison groups (e.g., drug A vs drug B) or dependent groups (e.g., the variable effects of drug A in mice with anxiety and depression).
4. Biases in data collection and statistical analysis: Similarly, while designing studies, it is crucial for the investigators to pay attention to control groups (conditions), randomization, blinding, and replication. By using a large sample size, randomization avoids unintentional bias and confounding errors. For example, one may wish to determine the impact of drug A on animal weight, heart rate, and body mass index. In this scenario, it is common to see investigators design separate experiments that are conducted separately. This approach introduces bias and is confounding. In contrast, the control group and the group receiving the drug can be randomized in sufficient numbers to oversee heart rate, BMI, and weight.
Similarly, post-hoc (or after-the-fact) analyses are not focused, and include multiple analyses to investigate potential relationships without full consideration of a suspected causal pathway. In such a scenario, investigators might be “fishing” for results where all potential relationships are analyzed. It is, thus, important to describe the methodology and rationale for using statistical tests to comply with the guidelines that are accepted as standards, such as the International Committee of Medical Journal Editors (ICMJE) guidelines.
The implications of statistical errors on manuscript publication process
Handling data correctly is important from the perspective of receiving accurate results. However, statistical soundness is also crucial from the perspective of getting published. If errors are spotted in the handling of statistics by the journals, the authors may be asked to make extensive changes or may even face rejection. Unfortunately, statistical errors are not that uncommon. Some of the most commonly occurring statistical errors during the publication stage are categorized as follows:
- Errors in study design (e.g., no randomization in controlled trials; inappropriate control group)
- Errors in data analysis (e.g., unpaired test for paired data; reporting P values without any other statistical data; using linear regression analysis without previous confirmation of linear relationship)
- Errors in data presentation (e.g., standard error instead of standard deviation to describe data; pie charts to present distribution of continuous variables; no adjustment for multiple comparisons )
- Errors in data interpretation (e.g., ‘caused if associate’ type of reasoning; interpretation of poorly done study as a well done one)
If errors are part of the description of the statistical analysis, revisions may be easy to incorporate. However, if the errors are made in data analysis, data interpretation, and discussion of the results, extensive changes would be required throughout the paper. In contrast, errors made in the study design often result in manuscript rejection as such errors cannot be corrected without repeating the whole study.
How the problem of misuse of statistics can be tackled
Statistical data is vital to newer and cutting-edge advancements in biomedicine. However, for this to happen there has to be a conscious effort to avoid misuse and abuse in gathering statistical data, analyzing it, and presenting it.
Researchers on their part need to be aware of and follow the accepted standards when it comes to handling statistics. The ICMJE mandates ‘Uniform Requirements for Manuscripts Submitted to Biomedical Journals’. These guidelines are recommendations to ensure correct application and explanation of statistical methods.
In addition to the ICMJE guidelines, researchers need to become aware of other available guidelines such as the ‘Statistical Analysis and Methods in the Published Literature’ (SAMPL) guidelines. These provide detailed recommendations on reporting of statistical methods and analyses by their type and are aimed to provide guidance in the design, execution, and interpretation of experimental studies.
Research papers in biomedicine are, in most cases, pillared on statistics. Therefore, most biomedical journals, especially the ones with a high impact factor, such as Lancet, Nature, Science, Cell, and JAMA employ biostatisticians among their associate editors and reviewers. This practice of including expert biostatisticians to evaluate manuscripts is now increasingly being adopted by several journals.
Admittedly, summarizing evidence and drawing conclusions based on data is challenging because of the number of variables in study designs, sample sizes, and outcome measures. The use of computers and statistical software tools has increased the ways in which data can be interpreted and analyzed. However, this also created room for more misinterpretations and errors.
As Dr. Jo Røislien, a Norwegian mathematician, biostatistician, and researcher in medicine, and an Associate Professor at the Department of Health Sciences, University of Stavanger, puts it, “[…]statistics quantifies the degree of certainty with which you should trust your results. Or not.” In conclusion, investigators should educate themselves about the best practices related to statistical methodology before beginning the study. While statistics is a powerful tool with which researchers can extend our current knowledge in biomedicine, only wielding this tool correctly will yield the desired output.
Would you like a 1:1 consultation with a biostatistician for assistance with power calculations and other statistical tests? Check out Editage’s Statistical Analysis & Review Service
Related reading:
Published on: May 21, 2018
Comments
You're looking to give wings to your academic career and publication journey. We like that!
Why don't we give you complete access! Create a free account and get unlimited access to all resources & a vibrant researcher community.
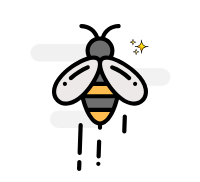
Subscribe to Conducting Research