Avoiding overfitting in biomedical research: a guide for researchers
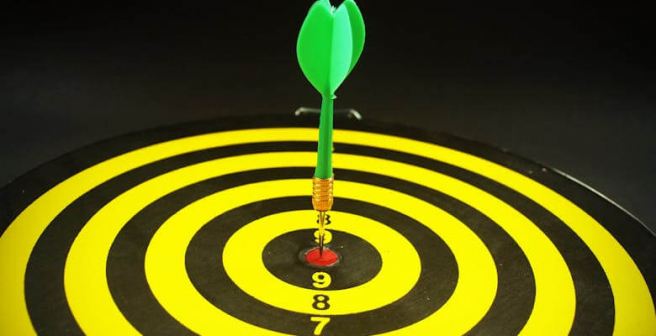
As you delve into the world of data analysis, you might encounter a sneaky adversary known as overfitting. In statistics, model overfitting refers to a scenario where a statistical model learns the training data too well, capturing noise or random fluctuations rather than the underlying pattern or relationship. This results in a model that performs well on the training data but fails to generalize to new, unseen data. Researchers often face the challenge of overfitting when developing predictive models or analyzing data. But don’t worry; we’re here to help you navigate this challenge and ensure your statistical models are rock-solid.
Understanding Overfitting
Imagine you’re trying to figure out a cake recipe, but besides thinking about the number of eggs to be used, you’re also obsessing about the number of sprinkles on top. That’s what overfitting does to your statistical models. It’s like memorizing the answers to a specific set of questions without truly understanding the underlying concepts. Your model “learns” the training data so well that it fails to generalize to real-world scenarios. Overfitting can lead to poor predictive performance and erroneous conclusions when applied to real-world scenarios.
Why Overfitting Matters
Overfitting might seem harmless at first, but it can wreak havoc on your research outcomes. Think of it as wearing glasses with the wrong prescription – everything looks fine up close, but you’re missing the bigger picture. In biomedical research, this could lead to faulty conclusions and unreliable predictions.
Strategies to Combat Overfitting
- Cross-validation: Split your data into multiple subsets, train your model on some, and evaluate it on the rest. This helps gauge how well your model generalizes to new data.
- Regularization: Add a penalty term to your model to discourage complexity. It’s like adding guardrails to keep your model from veering off course.
- Feature Selection: Choose your features wisely. Just like assembling a team, pick the best players (features) that contribute meaningfully to your model’s performance.
- Simplify Complexity: Keep it simple! Sometimes, a straightforward model can outperform a fancy one. Don’t overcomplicate things if you don’t have to.
- Data Augmentation: If your dataset is on the smaller side, consider beefing it up with bootstrapping or synthetic data generation. More data means a clearer picture for your model to learn from.
- Choose the Right Metrics: Use evaluation metrics like accuracy, precision, and recall to assess your model’s performance. It’s like giving your model a report card – grades matter!
Conclusion
Overfitting might seem like a formidable foe, but armed with the right strategies, you can conquer it. Remember, in the world of biomedical research, robust statistical models are your best allies. So, keep your models lean, mean, and ready to tackle any challenge that comes your way.
Unsure of how to tackle overfitting and other statistical challenges? Consult an expert biostatistician, under Editage’s Statistical Analysis & Review Services
Comments
You're looking to give wings to your academic career and publication journey. We like that!
Why don't we give you complete access! Create a free account and get unlimited access to all resources & a vibrant researcher community.
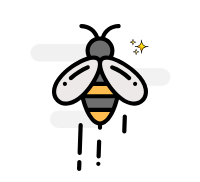
Subscribe to Conducting Research