Optimizing research quality: Importance of statistical power and how to calculate it in biomedical sciences
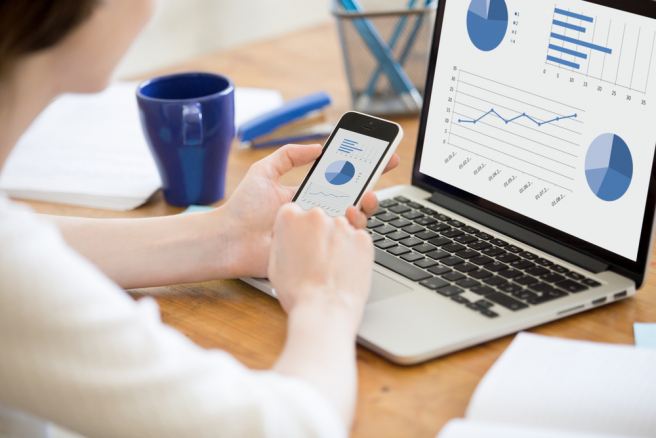
One of the key reasons for poor-quality research in the biomedical sciences is the lack of statistical power.[1] Many widely followed reporting guidelines like CONSORT (Consolidated Standards of Reporting Trials)[2] require authors to justify sample size. Journals like the British Journal of Surgery[3] and JAMA Neurology[4] require power calculations to be clearly stated in the manuscript. Others, like Molecular Genetics and Metabolism, clearly state that “[s] ubmitted manuscripts without a power calculation will be rejected and returned to authors without review.”[5] And it’s not just medical and life science journals that are strict about statistical power—the American Psychological Association also strongly recommends reporting a power analysis in the methods section of psychology papers, in its Reporting Standards for Research in Psychology[6].
What is statistical power?
In statistics, power refers to the ability of your study to identify effects of substantial interest. Underpowered studies often fail to detect important effects or end up with false-positive results, ultimately leading to inconsistent or misleading data and undermining the reliability of research in general.[7]
How do I calculate statistical power?
At the time of designing your study, you need to consider four essential factors:
1. Sample size, i.e., the number of units (e.g., patients), usually represented as “N.”
2. Size of the effect that you are interested in (usually, if you are looking for a large effect, you don’t need as big a sample as you would if you were looking for a small effect)
3. Alpha level: This is your significance threshold (it can be .001, .05, or .1). If your p values are at or above this level, you say that your result is not statistically significant.
4. Power: This is a value representing the likelihood of you finding an effect.
The above four parameters are interrelated, so if you have the values for three of them, you can calculate the value of the fourth. But usually, the alpha level is fixed (you generally have to choose between .001, .05, and .1) and by reviewing the literature, you will know roughly how large or small your effect can possibly be (effect size). So if you want your study to have good power, you will need to focus on sample size.
You’ll notice that there has been no mention of methodology in the above explanation. This is because the power of a study is independent of the methodology. You can conduct the most rigorous tests, such as randomized clinical trials even if your study has low statistical power. But by ignoring statistical power, you may end up wasting time and resources on a study that can’t produce sufficiently reliable and reproducible results since your sample size is too small for you to appropriately detect the effects you have chosen to study.
When should I calculate power?
Unfortunately, it’s very difficult to fix power after you have completed data collection. It’s therefore important to perform an a priori power calculation, to verify whether your study design has sufficient power. Long-term studies may also require interim power calculations so that you can adjust sample sizes accordingly and avoid both premature ending and unnecessary prolongation of the research. At times, you may also need to perform an a posteriori power analysis in order to understand the reason for a non-significant result. Power calculations are also valuable additions to your grant proposal, so that the funding agency’s reviewers can gauge the robustness of your study.
Conclusion
Power analysis is an important tool to maximize your study’s success in answering your research questions. Estimating the correct sample size required is necessary for producing high-quality and robust evidence.
Would you like a 1:1 consultation with a biostatistician for assistance with power calculations and other statistical tests? Check out Editage’s Statistical Analysis & Review Service
Published on: Feb 17, 2023
Comments
You're looking to give wings to your academic career and publication journey. We like that!
Why don't we give you complete access! Create a free account and get unlimited access to all resources & a vibrant researcher community.
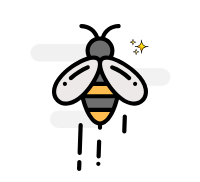
Subscribe to Conducting Research