Analyzing time-to-event data: What biomedical researchers need to know
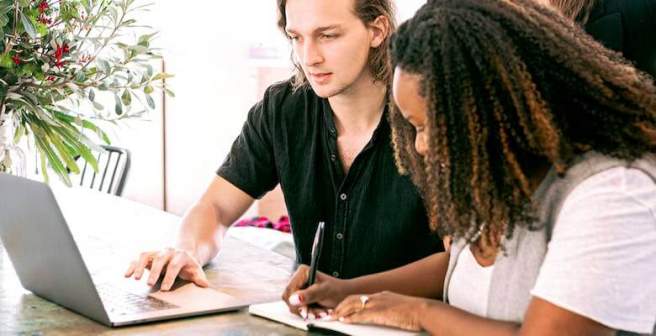
Time-to-event data, also known as survival data or time-to-failure data, is commonly encountered in biomedical research when studying the time it takes for an event of interest to occur. This event could be a patient's death, disease recurrence, the onset of a specific symptom, or any other event with a well-defined start and endpoint. Analyzing time-to-event data is crucial in understanding the progression and outcomes of diseases, treatment effectiveness, and more. Here are some important considerations for statistical analysis of time-to-event data in biomedical research:
Censoring
One of the most critical issues with time-to-event data is censoring. Censoring occurs when the event of interest has not yet occurred for some study participants at the time of analysis. These participants are still being followed, and their event times are unknown. Different types of censoring include right-censoring (most common), left-censoring, and interval-censoring. Statistical methods must account for censoring appropriately.
Survival Analysis
Survival analysis techniques, such as Kaplan-Meier curves, Cox proportional hazards model, and parametric survival models, are commonly used for analyzing time-to-event data. These methods are specifically designed to handle censored data and estimate survival probabilities and hazard rates.
It's important to test the assumptions underlying survival analysis, such as the proportionality assumption in the Cox model. If these assumptions are violated, alternative models or techniques may be required.
Hazard Function
The hazard function represents the probability of an event occurring at a specific time, given that it has not occurred before. It's a fundamental concept in survival analysis and can provide insights into the instantaneous risk of the event.
Stratification
In some cases, it's important to consider stratification, where you group participants based on specific characteristics (e.g., gender, age, treatment group) and analyze survival within these strata. Stratification helps account for potential confounding variables.
Covariates
In biomedical research, you often have covariates (e.g., genetic markers) that can influence survival. The Cox proportional hazards model is a widely used method for analyzing how these covariates impact survival.
Sample Size
The sample size in time-to-event studies is crucial, especially when events are rare. Inadequate sample sizes can lead to underpowered studies, making it challenging to detect significant differences.
Data Quality
Ensure data quality, including the accurate recording of event times and censoring information, to minimize bias in the analysis.
Reporting
When reporting results, provide clear summaries of survival curves, hazard ratios, confidence intervals, and p-values. Interpretation should be in the context of the specific research question.
Time-Dependent Covariates
Some factors may change over time (e.g., biomarker levels). Time-dependent covariates should be appropriately handled in the analysis.
Conclusion
In summary, the statistical analysis of time-to-event data in biomedical research is a specialized area that requires careful consideration of censoring, appropriate statistical techniques, and a deep understanding of underlying assumptions. It’s essential to analyze such data carefully in order to draw meaningful conclusions in studies involving survival and event occurrences.
Looking for expert advice on how to handle time-to-event data? Consult an experienced biostatistician under Editage’s Statistical Analysis & Review Services.
Comments
You're looking to give wings to your academic career and publication journey. We like that!
Why don't we give you complete access! Create a free account and get unlimited access to all resources & a vibrant researcher community.
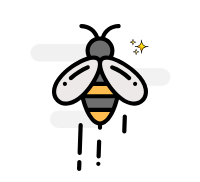
Subscribe to Conducting Research