Identifying biomarkers from Omics data: The role of statistics
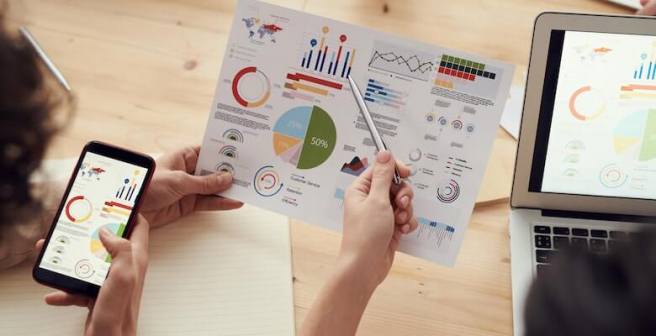
The emergence of omics technologies has revolutionized our understanding of diseases and opened up new possibilities for personalized medicine. The omics revolution encompasses a range of technologies, including genomics, transcriptomics, proteomics, metabolomics, and epigenomics. These technologies generate vast amounts of highly complex data.
One of the key challenges in omics research is identifying biomarkers—those elusive molecular signatures that can predict disease outcomes and guide treatment decisions. Biomarkers allow researchers to diagnose diseases, monitor treatment responses, and predict patient outcomes. With the help of omics data, researchers can identify biomarkers that are specific to certain diseases, subtypes, or stages, and thus develop more precise and personalized interventions.
In this blog post, we will look at the crucial role that statistics plays in the identification of biomarkers from omics data, and get an idea of the powerful tools and methods that are currently being used for this.
Preprocessing and quality control
Before running any kind of statistical analysis, it’s crucial to preprocess omics data. This involves removing technical artifacts, normalizing data across samples, and addressing missing values. For this, you can use statistical approaches such as imputation and outlier detection. Through preprocessing, you can ensure data integrity and reliability, thereby laying a solid foundation for subsequent analyses. For an overview of best practices in omics data preprocessing, you can look at Torres-Martos et al.’s (2023) case study, which draws on real data.i
Exploratory data analysis
Exploratory Data Analysis (EDA) is an indispensable step in identifying biomarkers. In EDA, researchers uncover patterns and structures within omics datasets by using various statistical techniques, such as principal component analysis (PCA), hierarchical clustering, and t-SNE. EDA provides valuable insights into the relationships between samples, identifies potential outliers, and highlights key features that may differentiate disease states or treatment responses. Various tools have been developed specifically for EDA, such as MetaOmGraphii and DanteRiii.
Differential expression analysis
Differential expression analysis is a statistical method used to identify genes, proteins, or metabolites that are significantly altered between different experimental conditions. Here, researchers use tests like the large-sample z test, ANOVA, or regression models to compare expression levels or abundances. In this way, they can identify which biomarkers are associated with specific phenotypes or clinical outcomes. Advanced methods like gene set enrichment analysis (GSEA) and pathway analysis provide a broader perspective by revealing the biological processes and pathways implicated in disease. If you’ve never used such methods before, Reimand et al. (2019) provide a practical step-by-step guide to pathway enrichment analysis, including a protocol designed for biologists with no prior bioinformatics training.iv
Machine learning and predictive modeling
Machine learning is utilized to identify biomarkers from omics data by employing algorithms that can analyze high-dimensional datasets and discover patterns and relationships. Techniques such as random forests, support vector machines, and neural networks are trained on labeled data to classify or predict disease outcomes. When you use machine learning, it’s important to exercise caution during feature selection, cross-validation, and model interpretation in order to prevent overfitting (i.e., the model learns the training data so well that it becomes overly specific and fails to generalize well to unseen data). Reel et al. (2021) provide a comprehensive review of different machine learning approaches for multi-omics data analysis, including recommendations specifically for interdisciplinary research.v
Validation and reproducibility
Biomarkers identified from omics data must undergo rigorous validation to ensure their robustness and reproducibility. Here, researchers use statistical techniques like cross-validation, bootstrapping, and permutation testing to assess whether biomarker signatures are accurate and effective across independent datasets or patient cohorts. Spratt and Ju (2016) provide a detailed guide to validating biomarker candidates.vi
Conclusion
The statistical analysis of omics data to identify suitable biomarkers can be complex. In order to identify robust biomarkers, it’s important to handle the data carefully and preferably include an experienced biostatistician in every stage of the research, so that everyone has a better understanding of the resultant data and its implications.
Do you want to unlock new insights from omics data and speed up biomarker discovery? Get the help of an experienced biostatistician through Editage’s Statistical Analysis & Review Services!
Comments
You're looking to give wings to your academic career and publication journey. We like that!
Why don't we give you complete access! Create a free account and get unlimited access to all resources & a vibrant researcher community.
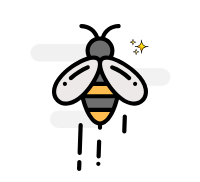
Subscribe to Conducting Research