12 Key precautions for biomedical researchers analyzing longitudinal data
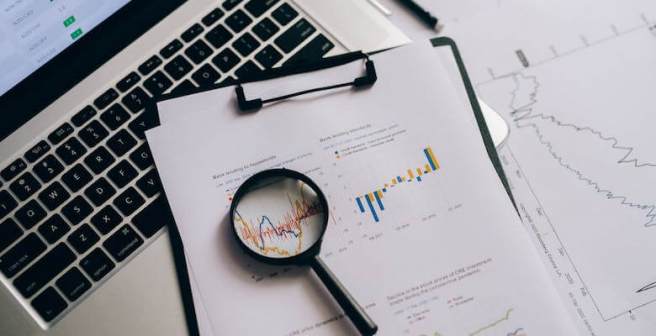
Longitudinal studies play a critical role in understanding the dynamics of health and disease over time. These studies involve the collection of data from the same subjects at multiple time points, making data analysis a complex and nuanced process. To ensure the validity and reliability of your findings, it's essential to take specific precautions during statistical analysis. Here are 10 key precautions for biomedical researchers conducting longitudinal studies:
- Data Quality Control: Implement rigorous data quality control measures to address issues like missing data, outliers, and inconsistencies. Data cleaning is crucial for maintaining data integrity.
- Select Appropriate Statistical Techniques: Choose statistical methods that are suitable for longitudinal data, such as mixed-effects models, generalized estimating equations, or autoregressive models. Using the wrong methods can lead to biased results.
- Longitudinal Data Structures: Recognize the different data structures in longitudinal studies, such as unbalanced, balanced, or irregularly spaced data. Your analysis plan should accommodate these structures.
- Account for Time: Time is a critical factor in longitudinal studies. Consider time as a covariate, and assess time trends and patterns within your data. This allows you to explore how outcomes change over time.
- Handling Missing Data: Develop a strategy for handling missing data, whether through imputation or other techniques. Be transparent about your approach in your research report to ensure reproducibility.
- Multiple Comparisons: Be cautious about multiple comparisons. Adjust significance levels or use methods like the Bonferroni correction to account for the increased risk of Type I errors when analyzing data at multiple time points.
- Control for Confounders: Identify potential confounding variables that may influence your results. Include these in your models to ensure the validity of your findings.
- Explore Interactions: Investigate interactions between variables, especially the interaction between predictors and time. This can reveal how the relationships change over the course of the study.
- Model Assumptions: Check the assumptions of the chosen statistical models, such as linearity, independence, and homoscedasticity. Violations of these assumptions can affect the validity of your results.
- Robustness Checks: Conduct sensitivity analyses to assess the robustness of your findings. This involves testing different models or approaches to ensure the consistency of results.
- Data Visualization: Use data visualization techniques to explore your data before, during, and after analysis. This helps identify trends, outliers, and potential issues that may require further investigation.
- Transparent Reporting: Document your analytical procedures thoroughly and report all relevant details in your research papers. Transparency is crucial for reproducibility and peer review.
Looking for a trusty collaborator to help you design a longitudinal study and analyze the data? Check out Editage’s Statistical Analysis & Review Services.
Comments
You're looking to give wings to your academic career and publication journey. We like that!
Why don't we give you complete access! Create a free account and get unlimited access to all resources & a vibrant researcher community.
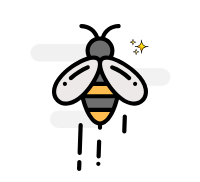
Subscribe to Conducting Research