A handy guide to Individual Participant Data Meta-Analysis
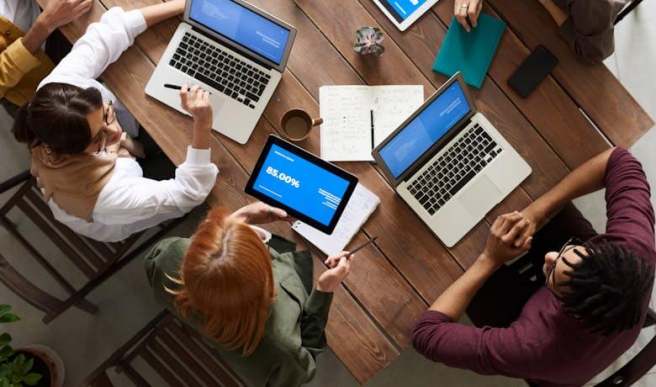
As vast amounts of data are published each year, scientists are constantly seeking innovative methods to extract meaningful insights from such data. One method that has gained increasing attention is Individual Participant Data Meta-Analysis (IPD-MA). IPD-MA combines raw data from individual participants across multiple studies. Unlike traditional meta-analyses, it looks at detailed data rather than just summaries. This helps find more precise answers, understand differences between studies, and make better decisions in fields like medicine and healthcare. In this blogpost, we’ll delve into the intricacies of IPD-MA, its advantages and limitations, and key statistical considerations for researchers venturing into this realm.
What is Individual Participant Data Meta-Analysis?
In essence, IPD-MA involves the pooling of raw data from individual participants across multiple studies, rather than relying solely on aggregated summary statistics. This granular approach offers several distinct advantages:
Pros of Individual Participant Data Meta-Analysis
- Increased Statistical Power: By combining data from multiple studies, IPD-MA enhances statistical power, enabling researchers to detect smaller effects or associations that may be missed in traditional meta-analyses.
- Exploration of Heterogeneity: With access to individual-level data, researchers can explore sources of heterogeneity more effectively, allowing for more nuanced subgroup analyses and the identification of potential effect modifiers.
- Detailed Covariate Adjustment: IPD-MA enables comprehensive adjustment for covariates at the individual level, reducing the risk of confounding and providing more accurate estimates of associations.
- Standardization and Harmonization: Through careful data harmonization, IPD-MA facilitates standardization of variables and methods across studies, ensuring consistency and comparability in the analysis.
- Flexibility in Analytical Approaches: Researchers have greater flexibility to employ advanced statistical techniques tailored to the specific research question, such as time-to-event analyses, longitudinal modeling, or propensity score matching.
Cons of Individual Participant Data Meta-Analysis
- Data Accessibility and Availability: Accessing individual participant data from multiple studies can be challenging, requiring collaboration with study authors, adherence to data-sharing policies, and addressing privacy and ethical considerations.
- Data Harmonization Challenges: Harmonizing data across studies may be labor-intensive and complex, particularly when dealing with disparate data formats, measurement scales, or variable definitions.
- Resource Intensiveness: Conducting an IPD-MA demands significant resources in terms of time, expertise, and computational power, from data collection and cleaning to analysis and interpretation.
- Potential for Bias: Despite rigorous quality control measures, the risk of bias due to selective data reporting, measurement error, or unmeasured confounding factors remains a concern in IPD-MA.
Key Statistical Considerations for Individual Participant Data Meta-Analysis
- Data Cleaning and Quality Control: Thorough data cleaning is essential to identify and address errors, missing values, and inconsistencies across datasets. Robust quality control measures are crucial to ensure the reliability and validity of the findings.
- Accounting for Study Heterogeneity: Assessing and quantifying heterogeneity between studies is paramount in IPD-MA. Statistical techniques such as meta-regression, subgroup analyses, and sensitivity analyses can help elucidate sources of heterogeneity and their impact on the results.
- Handling Missing Data: Strategies for handling missing data should be carefully considered, ranging from complete case analysis to imputation methods such as multiple imputation or maximum likelihood estimation.
- Addressing Confounding and Bias: Comprehensive adjustment for potential confounders is essential to minimize bias and enhance the internal validity of the analysis. Sensitivity analyses can assess the robustness of the findings to different modeling assumptions.
- Publication Bias and Small-Study Effects: Vigilance against publication bias and small-study effects is critical in IPD-MA. Techniques such as funnel plots, Egger’s regression, or trim-and-fill methods can help detect and account for asymmetry in the data.
Conclusion
In conclusion, IPD-MA is a powerful tool for integrating evidence across diverse studies in biomedical research. While offering unparalleled insights into complex phenomena, IPD-MA also presents unique challenges and considerations that researchers must navigate with care and expertise. By embracing rigorous methodologies, transparent reporting practices, and collaborative efforts, biomedical researchers can harness the full potential of IPD-MA to drive scientific discovery and improve patient outcomes.
Eager to explore the fascinating world of IPD-MA? Take the help of an experienced biostatistician, under Editage’s Statistical Analysis & Review Services