An introduction to Bayesian Information Borrowing for biomedical researchers
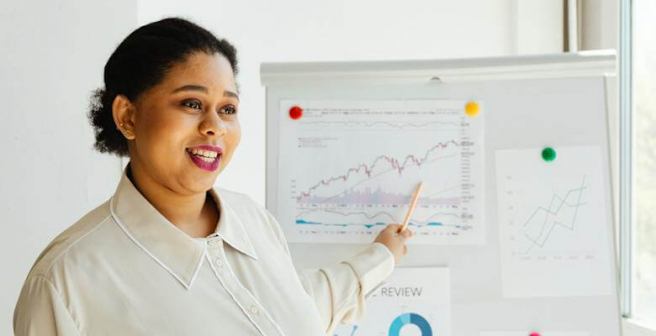
In biomedical research, you often have limited data for a specific study, which can lead to uncertainty in your conclusions. Bayesian information borrowing allows you to leverage existing knowledge from related studies or domains. This can help in situations where individual datasets might be small or noisy.
What is Bayesian Information Borrowing?
Bayesian information borrowing is a statistical concept that can be valuable for biomedical researchers. It involves combining information from multiple sources, such as different studies or data types, to improve the accuracy and reliability of statistical analyses.
For instance, if you're studying a rare disease and have limited patient data, Bayesian information borrowing enables you to incorporate information from other studies on similar diseases. By doing so, you can make more informed inferences, estimate parameters with greater precision, and enhance the power of your analyses.
The key idea behind Bayesian information borrowing is to create a framework that allows data from multiple sources to inform each other. Bayesian statistics, with its flexibility in handling uncertainty, is well-suited for this purpose. Researchers can define prior beliefs about parameters and update them as new data is incorporated, resulting in more robust and accurate conclusions.
Steps in Bayesian Information Borrowing
- Define the Research Problem: Clearly articulate the biomedical research question you want to address and identify the data sources relevant to your study.
- Select Bayesian Models: Choose appropriate Bayesian models for each data source. These models should represent the underlying biology or phenomena you're investigating. For instance, you might use different models for gene expression data, clinical outcomes, or genetic markers.
- Specify Priors: Define prior distributions for the parameters in your models. Priors can be informed by existing knowledge, expert opinions, or non-informative if little prior information is available.
- Data Integration: Combine data from various sources into a single analysis. Ensure that data harmonization and preprocessing are performed accurately to account for differences in scales, units, and other data-specific issues.
- Bayesian Inference: Use Bayesian methods to estimate model parameters. This involves computing posterior distributions for the parameters based on the combined data and prior information.
- Model Validation: Assess the validity and performance of your Bayesian models. Techniques like cross-validation can help evaluate model fit and predictive accuracy.
Precautions to take for Bayesian Information Borrowing
- Data Quality Assurance: Ensure that data from different sources are of high quality and relevance. Data errors or biases can lead to incorrect inferences.
- Model Assumptions: Be aware of the assumptions underlying your Bayesian models. Violating these assumptions can lead to biased results.
- Priors Sensitivity: Be cautious about the choice of prior distributions. The results can be sensitive to the prior information, so conduct sensitivity analyses to evaluate the impact of different priors.
- Data Heterogeneity: Recognize the potential heterogeneity among data sources. Biases, confounding factors, or differences in study design should be carefully considered in the analysis.
- Transparency and Reporting: Clearly document the methods, models, and prior information used. Transparency is critical for reproducibility and for other researchers to evaluate your work.
- Cross-Validation: Use cross-validation and other validation techniques to assess the performance of your models. This helps identify potential issues with overfitting or underfitting.
- Expert Involvement: Engage with both experts in the biomedical field and biostatisticians versed in Bayesian statistics. Collaboration with domain experts can lead to better-informed model choices and prior specifications.
Conclusion
In summary, Bayesian information borrowing involves integrating data from multiple sources using Bayesian methods. Bayesian information borrowing is a powerful tool in biomedical research. By pooling information from various sources, you can increase the reliability of your findings, especially when you’ve got limited data.
Transparency and expert involvement play a crucial role in Bayesian analysis. Get advice from an expert biostatistician under Editage’s Statistical Analysis & Review Services.
Comments
You're looking to give wings to your academic career and publication journey. We like that!
Why don't we give you complete access! Create a free account and get unlimited access to all resources & a vibrant researcher community.
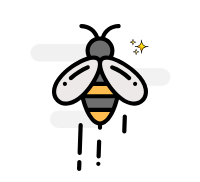
Subscribe to Conducting Research