Conducting a longitudinal study? Here’s why and how you should use Bayesian methods
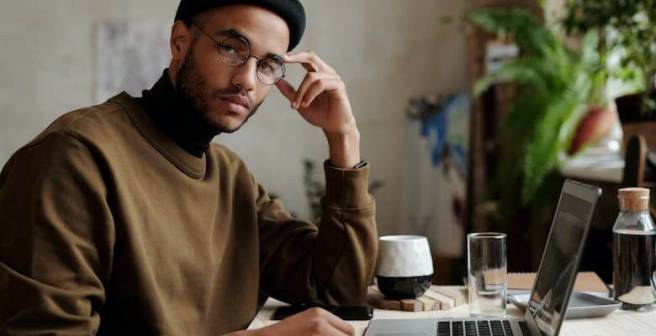
Longitudinal studies in biomedical research are crucial for unraveling the dynamic nature of health and disease over time, allowing researchers to track the progression of conditions, identify risk factors, and assess the effectiveness of interventions. However, they also come with unique challenges related to data analysis: addressing temporal dependencies, handling missing data, and capturing individual variability over time. Thus, longitudinal studies require sophisticated statistical methods for robust and accurate inference. This is where Bayesian methods can be particularly valuable.
Why Use Bayesian Methods to Analyze Longitudinal Data?
Bayesian methods offer solutions to several challenges in longitudinal studies, enhancing the robustness and flexibility of analyses. Firstly, handling missing data is a common challenge in longitudinal research. Bayesian approaches can naturally accommodate missing data through techniques like multiple imputation, allowing for more accurate and unbiased parameter estimates.
Secondly, Bayesian methods excel in modeling complex and hierarchical structures inherent in longitudinal data. They efficiently capture individual variability, account for correlations within repeated measurements, and distinguish between fixed and random effects. This flexibility is crucial in understanding the nuanced dynamics of biomedical processes over time.
Thirdly, Bayesian methods provide a framework for incorporating prior information, aiding in cases where limited sample sizes may lead to uncertainty. This is especially beneficial in biomedical research, where prior knowledge or expert opinions can enhance the precision of parameter estimates.
Moreover, Bayesian model selection techniques, such as Bayesian model averaging (BMA) and the deviance information criterion (DIC), can be used to choose the most appropriate model among competing models. Depaoli et al. (2021) provide a detailed explanation of how BMA can be used for model selection.
Finally, Bayesian techniques are adept at handling nonlinear relationships and diverse data types within the same study, offering a unified framework for analyzing heterogeneous longitudinal datasets. This ability is crucial when studying complex biological systems with intricate temporal dynamics.
Bayesian Methods That Work Well in Longitudinal Studies
Let’s now take a look at various Bayesian methods that can be used in longitudinal studies:
- Hierarchical Models: Bayesian hierarchical models allow for the incorporation of various sources of variability and are particularly useful when data are collected at multiple levels, such as repeated measurements within individuals and individuals within different groups. These models can account for individual variation while also estimating population-level parameters. Recently, Chen and Hsiao (2023) showed how Bayesian hierarchical models can be useful in clinical trials with alternative designs, such as basket trials.
- Mixed Effects Models: Bayesian mixed effects models are used to account for both fixed and random effects in longitudinal data analysis. They are suitable for modeling individual-specific variability and capturing correlations within repeated measurements. For instance, Baurley et al. (2022) used a combination of Bayesian mixed effects models and machine learning to understand behavior patterns in substance abuse disorder and thus develop individualized interventions.
- Joint Modeling: In longitudinal studies, it is common to have multiple types of data, such as clinical outcomes and time-to-event data. Bayesian joint models can simultaneously analyze these different data types, allowing for the estimation of associations between them. Recently, Bean et al. (2023) proposed a Bayesian joint modeling approach that can be used in multi-region clinical trials.
- Nonlinear Models: In some biomedical studies, nonlinear relationships may exist between variables over time. Bayesian methods can handle nonlinear models and help capture complex dynamics within the data.
- Bayesian Structural Equation Modeling (BSEM): BSEM is a Bayesian extension of structural equation modeling that can be applied to longitudinal data. It allows researchers to test complex theoretical models and relationships between variables over time. See, for example, how Vasantha et al. (2021) used BSEM to study post treatment health-related quality of life among tuberculosis patients.
- Time-Series Analysis: For time-dependent data in longitudinal studies, Bayesian time-series models can be employed to capture temporal dependencies and make predictions about future measurements. Batley et al. (2020) have described in detail how Bayesian time-series models can be used especially for small samples, such as in trauma research.
- Survival Analysis: In longitudinal studies with time-to-event data, Bayesian survival analysis can be used to estimate survival curves, hazard ratios, and other relevant quantities. You can refer to Bartoš et al. (2022) for further tips on running Bayesian survival analysis.
Conclusion
Bayesian methods are increasingly popular in biomedical research, particularly for longitudinal studies, due to their flexibility in handling complex data and modeling assumptions. Using Bayesian methods in your longitudinal study can help you generate more robust and high-quality data, and thus uncover essential insights for improving patient outcomes and healthcare strategies.
Looking for guidance on how to use Bayesian methods in your research? Consult an expert biostatistician under Editage’s Statistical Analysis & Review Services.
Comments
You're looking to give wings to your academic career and publication journey. We like that!
Why don't we give you complete access! Create a free account and get unlimited access to all resources & a vibrant researcher community.
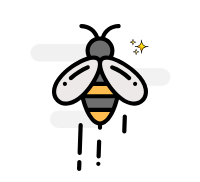
Subscribe to Conducting Research