The amazing benefits of Bayesian statistics in clinical trial design
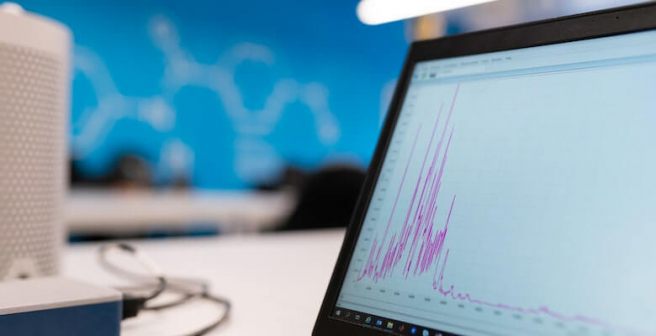
Clinical trials, especially randomized controlled trials, are considered the gold standard for demonstrating the efficacy of a new intervention. In a traditional clinical trial, researchers follow a fixed, pre-defined plan, often called a frequentist approach. They set specific criteria and sample sizes in advance and then collect data according to that plan. The analysis is typically based on frequentist statistics. This type of trial design is relatively simple to use as power can be calculated using a standard formula; however, in such trials, researchers may have to recruit an unnecessarily large sample. This poses a burden on both patients and the researchers.
In contrast, Bayesian clinical trial design is more flexible and adaptive, enabling researchers to save time and resources by lowering the sample size required or by improving power. As Hong et al. (2022) found in a real-world oncology trial, Bayesian adaptive designs can substantially outperform a fixed design in terms of lower type I and II errors, and average sample size required for optimal power.
Bayesian Adaptive Designs: An Overview
Bayesian clinical trial design is an approach to planning and conducting clinical trials that relies heavily on Bayesian statistics. One of the key ways in which Bayesian statistics differs from traditional frequentist statistics is that Bayesian statistics allows researchers to make use of what they already know about the research topic. Here’s how Bayesian clinical trial design works:
Prior Information: Researchers start with prior information, which includes existing data and knowledge about the treatment or condition. This prior information could come from previous studies, expert opinions, or historical data.
Continuous Learning: As the trial progresses and new data is collected, Bayesian methods continuously update the analysis. This means that researchers can adapt the trial based on the emerging results. If the treatment shows promising results early on, the trial might be expanded. Conversely, if it’s not effective or safe, the trial can be stopped sooner to save resources.
Probabilistic Inferences: Bayesian statistics provide probabilistic inferences. Instead of saying a treatment is simply "effective" or "ineffective," it provides a probability distribution of the treatment’s effectiveness. This can give a more nuanced and informative picture of the treatment’s impact.
Optimization: Bayesian trial design allows for the optimization of various aspects, such as sample size, treatment dosage, or patient selection criteria, based on the evolving data. This can lead to more efficient and cost-effective trials.
Popular Bayesian Methods Used in Adaptive Clinical Trials
Bayesian methods enable researchers to make changes to various aspects of a clinical trial as new data becomes available. Here are some common Bayesian adaptive trial design techniques:
Bayesian Continual Reassessment Method (CRM): In dose-finding trials, CRM helps find the optimal dose by starting with a prior belief about the dose-response relationship and then updating it as each patient’s outcome is observed. It’s used to efficiently identify the right dosage with the fewest patients exposed to potentially ineffective or toxic levels.
Bayesian Response-Adaptive Randomization: This method dynamically adjusts the allocation of patients to different treatment arms based on the observed responses. If one treatment appears more effective, more patients may be assigned to that arm during the trial, increasing the chances of detecting treatment differences sooner.
Bayesian Sample Size Re-Estimation: Researchers can periodically re-estimate the required sample size during the trial based on the accumulated data. If the observed treatment effect is larger or smaller than expected, the sample size may be adjusted to maintain adequate statistical power or save resources.
Bayesian Predictive Probability: This approach calculates the probability of achieving a specific outcome (e.g., treatment success) based on the current trial data. If the probability exceeds a predefined threshold, the trial may be stopped early for success or futility.
Bayesian Hierarchical Models: These models allow for borrowing strength across different parts of the trial, such as different study sites or patient subgroups. Information from one group can inform decisions about another, leading to more efficient use of data.
Bayesian Sequential Testing: Unlike traditional fixed-sample designs, Bayesian sequential testing allows for ongoing monitoring and potential early stopping for efficacy or futility, depending on the emerging results.
Bayesian Decision-Theoretic Designs: These designs consider not only the statistical aspects of a trial but also the clinical and economic consequences of different decisions. They aim to find the optimal decision strategy that maximizes the overall benefit.
Drawbacks of Bayesian Adaptive Designs
While Bayesian adaptive clinical trial designs offer flexibility and potential efficiency gains, they also come with challenges. Let’s take a look at the most common ones:
Complexity: Bayesian adaptive trials can be more complicated to plan and manage compared to traditional trials. They require advanced statistical expertise and careful design, which can increase the complexity and cost of the study.
Regulatory Challenges: Some regulatory agencies may be less familiar with Bayesian methods, which can lead to challenges in getting trial designs approved. Researchers may need to spend more time explaining and justifying their approach.
Risk of Bias: If not conducted rigorously, there’s a risk of introducing bias into the trial. The flexibility of Bayesian designs means that researchers can adapt the trial based on emerging data, but this adaptability must be carefully controlled to avoid introducing bias.
Data Dependency: Bayesian trials heavily rely on the initial assumptions and prior information. If these assumptions are incorrect or the prior data is unreliable, it can lead to inaccurate conclusions about the treatment’s effectiveness.
Resource Intensive: Bayesian trials may require more resources, especially in terms of computational power and data management. This can make them more expensive and potentially less accessible, particularly for smaller research teams.
Communication Challenges: Explaining the results of Bayesian trials to non-experts, including patients and the public, can be more challenging due to the probabilistic nature of Bayesian statistics. It may require more effort to convey the results in a clear and understandable way.
Ethical Considerations: Adaptive trials raise ethical questions, especially when it comes to patient consent. Patients might not be comfortable with the idea that the trial design could change while they’re participating, potentially impacting their treatment.
Conclusion
In summary, Bayesian adaptive clinical trial design is about using prior knowledge, adapting to new data as it becomes available, and making probabilistic inferences to optimize the trial process. It offers greater flexibility and the potential to make more informed decisions during the course of the trial, ultimately improving the efficiency of clinical research.
Our experienced biostatisticians can guide you through complex Bayesian analyses at any stage of your research journey. Check out Editage’s Statistical Analysis & Review Services.
Comments
You're looking to give wings to your academic career and publication journey. We like that!
Why don't we give you complete access! Create a free account and get unlimited access to all resources & a vibrant researcher community.
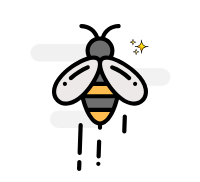
Subscribe to Conducting Research