Unlocking the benefits of Bayesian adaptive clinical trial design for medical and life science research
Clinical trial design is a critical component of medical and life science research, as it determines how new treatments and interventions are tested and evaluated. Traditionally, clinical trials have used frequentist statistical methods that rely on fixed sample sizes, predetermined endpoints, and strict hypothesis testing. However, these methods have several limitations, including inefficient use of resources and delays in drug development.
In recent years, there has been a growing interest in using Bayesian adaptive clinical trial design as an alternative approach. In this blog post, we'll explore what Bayesian adaptive designs are, how they work, and why they are becoming increasingly popular in medical and life science research.
Traditional clinical trial design
Traditional frequentist clinical trial designs have several limitations that can lead to inefficiencies and delays in drug development. One major limitation is the need for a fixed sample size, which can be challenging to determine accurately in advance. If the sample size is too small, the trial may be underpowered and fail to detect a real effect. Conversely, if the sample size is too large, resources may be wasted, and the trial may take longer than necessary to complete.
Another limitation of traditional designs is the use of predetermined endpoints, which can limit the ability to adapt the trial based on emerging data. In addition, frequentist methods typically rely on strict hypothesis testing and p-values, which can lead to dichotomous conclusions about the success or failure of a trial without taking into account the uncertainty and variability inherent in real-world data.
Bayesian adaptive clinical trial design
Bayesian adaptive clinical trial design is an alternative approach that allows trial parameters to be modified based on accumulating data. Bayesian methods use prior knowledge and experience to update the probability of hypotheses in light of new evidence. In clinical trial design, this means that as data is collected, the probability of treatment success can be continually updated, allowing for more efficient use of resources and potentially faster identification of effective treatments.
One example of Bayesian adaptive trial design is the use of Bayesian hierarchical models to estimate treatment effects in multi-arm trials. In these trials, multiple treatments are compared against each other using interim analyses, and the results are used to update the probability of treatment success for each treatment arm. This approach can result in a higher probability of identifying the most effective treatment arm early in the trial, reducing the overall study duration and cost.
Examples of Bayesian adaptive designs
Bayesian adaptive designs are increasingly used in medical research to efficiently and ethically answer research questions. Here are some examples:
Bayesian adaptive dose-finding studies: In phase I trials, investigators aim to identify the maximum tolerated dose (MTD) of a new drug. Bayesian adaptive designs can be used to adaptively modify the dose based on accumulating data, using prior information about the toxicity and efficacy of the drug.
Bayesian adaptive randomization: In clinical trials, randomization is used to assign participants to treatment groups. Bayesian adaptive randomization can be used to adjust the probability of treatment allocation based on the current state of evidence, such as early response rates or biomarker data.
Bayesian adaptive enrichment designs: In phase II trials, investigators may want to enroll only patients who are more likely to benefit from the experimental treatment. Bayesian adaptive enrichment designs can be used to adjust the eligibility criteria based on interim data, using prior information about the disease and treatment.
Bayesian adaptive seamless designs: In some cases, researchers may want to combine phases II and III trials to expedite drug development. Bayesian adaptive seamless designs can be used to adaptively modify the study design and sample size based on interim data, while controlling the overall type I error rate.
Criticisms and challenges
Despite the potential benefits of Bayesian adaptive clinical trial design, there are also criticisms and challenges associated with the approach. One challenge is the need for more complex statistical modeling, as Bayesian methods typically require the specification of prior distributions and the use of Markov Chain Monte Carlo (MCMC) methods. In addition, the interim analyses that are a key component of adaptive designs can introduce biases if not carefully controlled.
Would you like a 1:1 consultation with an expert statistician? Check out Editage’s Statistical Analysis & Review Service.
Comments
You're looking to give wings to your academic career and publication journey. We like that!
Why don't we give you complete access! Create a free account and get unlimited access to all resources & a vibrant researcher community.
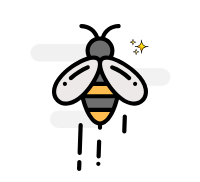
Subscribe to Conducting Research