Using bayesian methods for data cleaning: A guide for biomedical researchers
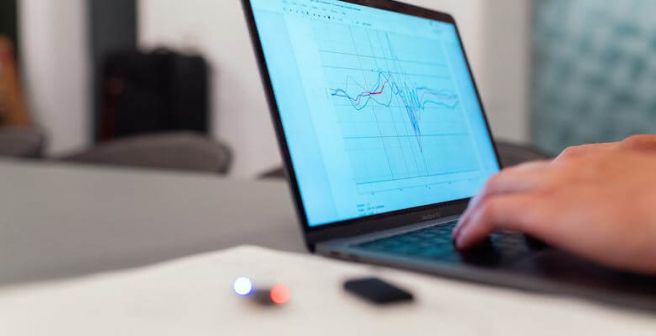
Are you tired of spending hours sifting through messy data, trying to separate the signal from the noise? Well, you're in luck because today, we're diving into the world of Bayesian methods and how they can be your secret weapon for efficient and effective data cleaning. So, grab your lab coat and let's get started!
What's Data Cleaning, Anyway?
Before we jump into the exciting world of Bayesian methods, let's make sure we're all on the same page. Data cleaning is like tidying up your room before guests arrive. In the realm of biomedical research, it's the process of getting rid of errors, inconsistencies, and outliers in your data. Clean data means more accurate results, which is what we all aim for.
The Bayesian Way: What Is It?
Bayesian statistics is a mathematical framework for modeling and updating beliefs about uncertain events or parameters. It combines prior knowledge and new data to calculate probabilities, allowing for more informed and flexible inference and decision-making.
Bayesian statistics provide a robust framework for handling the uncertainty and complexity often found in biomedical data. They allow researchers to explicitly model and account for uncertainties, which can lead to more accurate and reliable data cleaning processes and subsequently more valid and generalizable research results. These methods are particularly valuable when dealing with small sample sizes, complex data structures, or when prior domain knowledge is available to inform the analysis.
Why Use Bayesian Methods for Data Cleaning?
Dealing with Uncertainty: Biomedical data is often filled with uncertainty due to various sources of noise. Bayesian methods are brilliant at handling this uncertainty and making the data cleaner by providing probabilistic interpretations.
Flexibility: These methods allow you to build models that adapt to different data patterns. You're not locked into one fixed cleaning approach. It's like having a versatile toolbox.
Bayesian Methods for Data Cleaning
Here are some different Bayesian methods used for data cleaning in this field:
1. Outlier Detection:
Bayesian methods can be used to identify outliers in the data, which may be caused by measurement errors or other anomalies. Outliers can distort statistical analyses and lead to incorrect conclusions. One common approach is to use a Bayesian model to estimate the underlying distribution of the data and identify observations that have low posterior probabilities under this distribution.
2. Imputation of Missing Data:
In biomedical research, missing data is a common issue due to dropout, measurement errors, or other reasons. Bayesian methods can be used to impute missing values. By modeling the uncertainty associated with missing data, Bayesian imputation provides a more accurate estimation of parameters and better accounts for the uncertainty in the analysis.
3. Data Transformation:
Sometimes, data cleaning involves transforming variables [MR1] to meet the assumptions of statistical models. Bayesian statistics can be used to model the relationships between variables and apply transformations that optimize model fit and interpretability.
4. Model Selection:
Bayesian model selection techniques, such as Bayesian Information Criterion (BIC) or Bayes factors, can be used to determine the most appropriate model for the data. This helps in selecting the best-fitting model while avoiding overfitting.
5. Noise Reduction:
In some cases, data may be noisy due to factors like measurement errors. Bayesian approaches can incorporate prior information or constraints on parameters to reduce noise and improve the accuracy of data.
Conclusion
Bayesian methods provide a flexible framework for modeling uncertainties and making data-driven decisions, which can be particularly valuable in the context of biomedical research. This makes Bayesian statistics a powerful tool for data cleaning, ensuring you are working with high-quality data.
Ready to dive into the fascinating world of Bayesian statistics? Consult an expert biostatistician under Editage’s Statistical Analysis & Review Services.
Comments
You're looking to give wings to your academic career and publication journey. We like that!
Why don't we give you complete access! Create a free account and get unlimited access to all resources & a vibrant researcher community.
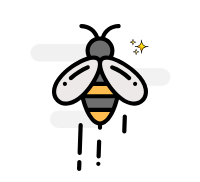
Subscribe to Conducting Research