Exploring high-dimensional data with multidimensional scaling: A handy guide for biomedical researchers
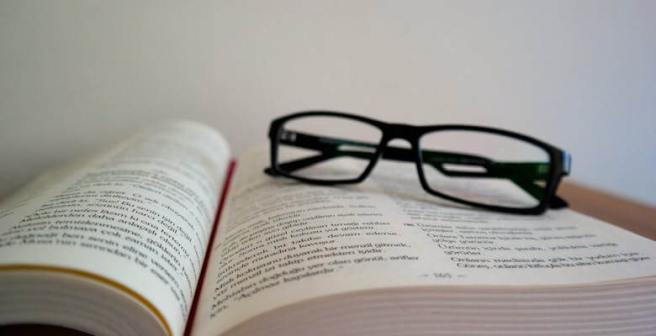
As biomedical researchers, we often find ourselves drowning in a sea of data. With the advancement of technology, we can now generate massive datasets containing information on genes, proteins, patient characteristics, and more. However, making sense of this wealth of information can be a daunting task. That’s where Multidimensional Scaling (MDS) comes into play – a powerful tool that can help us navigate through the complexity of high-dimensional data. For example, MDS has recently been used to reconstruct genomic DNA loops, analyze the relationship between intelligence and academic achievement, and explore the relationship between the relationship between electrogustometry and the whole mouth test.
What is Multidimensional Scaling (MDS)?
MDS is a statistical technique that allows us to visualize the underlying structure of complex datasets in a lower-dimensional space. In simpler terms, it helps us to see patterns and relationships in our data by representing it in a more manageable form. Hout et al. (2013) provide a detailed guide to MDS, including steps to address key issues that could arise during your analysis.
Visualizing Complex Relationships with MDS
One of the most significant advantages of MDS is its ability to reveal intricate relationships between data points. Imagine you have a dataset with hundreds or even thousands of variables – genes, proteins, clinical measurements, you name it. MDS can condense all this information into a comprehensible plot, where each data point represents a sample or observation, and the distances between points reflect their similarities or differences.
Dimensionality Reduction with MDS
Another key benefit of MDS is its dimensionality reduction capabilities. By projecting high-dimensional data onto a lower-dimensional space (typically 2D or 3D), MDS simplifies the data visualization process without losing essential information. This reduction in dimensionality makes it easier for researchers to interpret and analyze the data, leading to insights that may have been hidden in the original high-dimensional space.
Comparing and Contrasting Datasets with MDS
MDS also enables researchers to compare and contrast different datasets or subsets within a dataset. By visualizing multiple datasets on the same plot, researchers can quickly identify similarities and differences between groups, potentially uncovering valuable insights into disease mechanisms, treatment responses, or other biological phenomena.
Advantages of MDS over Other Methods
Compared to other techniques like Principal Component Analysis (PCA) or t-SNE (t-distributed Stochastic Neighbor Embedding), MDS offers several advantages:
- Preservation of Distances: MDS aims to preserve the pairwise distances or dissimilarities between data points as much as possible in the lower-dimensional space. This makes it particularly useful when the relationships between data points are best represented by distances rather than clusters or densities.
- Robustness to Outliers: MDS is less sensitive to outliers compared to some other dimensionality reduction techniques like PCA. Outliers have less influence on the final configuration of points in the MDS plot, allowing researchers to focus on the overall structure of the data.
Disadvantages and Limitations of MDS
While MDS is a powerful tool for visualizing high-dimensional data, it’s essential to acknowledge its limitations:
- Computational Complexity: MDS can be computationally intensive, especially for large datasets with many observations or variables. Researchers may need to optimize parameters or use parallel computing techniques to speed up the analysis.
- Interpretation Challenges: Interpreting MDS plots requires careful consideration of the underlying data and the chosen dissimilarity measure. Without a clear understanding of the data’s characteristics, misinterpretations or erroneous conclusions may arise.
Conclusion
In conclusion, Multidimensional Scaling (MDS) is a valuable tool for biomedical researchers seeking to explore complex relationships in high-dimensional data. By providing intuitive visualizations and facilitating dimensionality reduction, MDS empowers researchers to uncover hidden patterns and gain insights that can drive scientific discovery and improve patient outcomes. However, like any statistical technique, it’s essential to use MDS judiciously, considering its advantages, limitations, and appropriateness for the specific research question at hand.
Looking for support in handling high-dimensional data? Consult an experienced biostatistician under Editage’s Statistical Analysis & Review Services.
Comments
You're looking to give wings to your academic career and publication journey. We like that!
Why don't we give you complete access! Create a free account and get unlimited access to all resources & a vibrant researcher community.
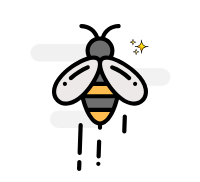
Subscribe to Conducting Research