4 Ways to make the most of your ANOVA: Uncovering the real differences between groups
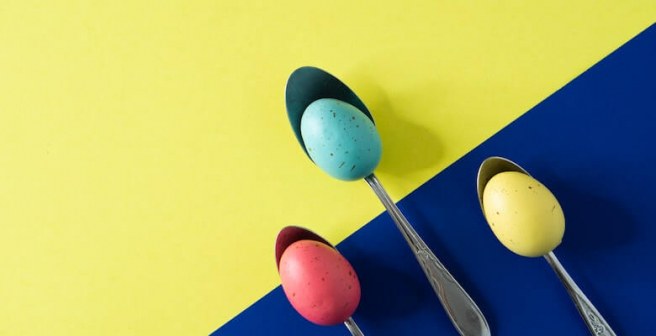
When you’ve got a bunch of different groups in your study, it can be tricky to know which test is the best for comparing differences between them. ANOVA is a powerful inferential statistical technique that allows us to compare means across multiple groups, providing valuable insights into experimental data. In this blog post, we’ll explore four key practices to maximize the potential of your ANOVAs.
Verify that the data meet the assumptions of an ANOVA
Before plunging into an ANOVA, it’s crucial to ensure that your data meet certain assumptions. These assumptions include
Normality: The data within each group should be normally distributed.
Homogeneity of variance: The variance of the data within each group should be equal.
Independence: The observations within each group should be independent.
Random sampling: Observations within each group have been sampled randomly and are independent of each other.
Violations of these assumptions can impact the validity of your results.
For example, suppose you’re conducting a study investigating the effect of a new drug on blood pressure. You collect blood pressure measurements from three groups: control, low dosage, and high dosage. To verify normality, you could create histograms of the blood pressure measurements for each group and visually inspect their distributions. Additionally, tests like the Shapiro-Wilk test can provide quantitative evidence of normality.
Remember, assessing assumptions upfront will help you avoid potential pitfalls and increase the reliability of your ANOVA results.
Calculate effect size
While p-values are commonly used in hypothesis testing, they only tell us if an effect is statistically significant or not. Effect size measures, on the other hand, provide valuable information about the magnitude and practical significance of the observed effects.
Let’s say you’re studying the impact of different exercise programs on body mass index (BMI) in a sample of obese individuals. After performing an ANOVA, you find a significant difference among the exercise groups. To better understand the strength of this effect, you can calculate eta-squared, which represents the proportion of total variance explained by the group differences.
By considering effect size measures, such as eta-squared, partial eta-squared, or omega-squared, you gain a deeper understanding of the practical significance of your findings, allowing you to communicate their importance more effectively.
Run post-hoc comparisons
An ANOVA helps us determine if there are any differences among the groups, but it doesn’t tell us which specific groups differ from each other. That’s where post-hoc comparisons come into play.
Suppose you’re investigating the effect of different drug treatments on cell viability in a cancer cell line. ANOVA reveals a significant overall difference among the treatment groups. To pinpoint the specific pairwise differences, you can use post-hoc tests like Tukey’s Honestly Significant Difference (HSD) or Bonferroni correction. These tests provide adjusted p-values for multiple comparisons, helping you identify which treatment groups significantly differ from one another.
Post-hoc comparisons provide a more detailed picture of the relationships between the groups, enabling you to draw more specific conclusions from your ANOVA results.
Check for interaction between variables
Interactions occur when the effect of one variable on the outcome depends on the level of another variable. Including interactions in your ANOVA can reveal more complex relationships between factors.
For instance, suppose you’re examining the effect of a nutritional intervention on cholesterol levels among male and female participants. Your ANOVA detects a significant main effect of the nutritional intervention, but it’s crucial to investigate if there’s an interaction between the nutritional intervention and gender. By including an interaction term in the ANOVA model and examining its significance, you can determine whether the effect of the intervention differs depending on gender.
Identifying and interpreting interactions is essential for fully understanding the complexities of your data and drawing accurate conclusions about the factors influencing your outcome.
Conclusion
By verifying data assumptions, calculating effect sizes, running post-hoc comparisons, and considering interactions, you can unlock deeper insights from your ANOVA results and make them more robust. Remember, ANOVA is a valuable tool, but it’s just one piece of the statistical puzzle. Continually expanding your knowledge of statistical methods will empower you to make informed decisions and contribute to groundbreaking discoveries in the world of biomedical research.
Need help in assessing the differences between groups in your study? Our expert biostatisticians can help you choose, run, and interpret the results of the right test. Check out Editage’s Statistical Analysis & Review Services.
Comments
You're looking to give wings to your academic career and publication journey. We like that!
Why don't we give you complete access! Create a free account and get unlimited access to all resources & a vibrant researcher community.
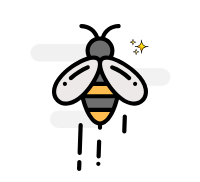
Subscribe to Conducting Research