Analyzing variability in biomedical research data: Understanding heteroscedasticity and homoscedasticity
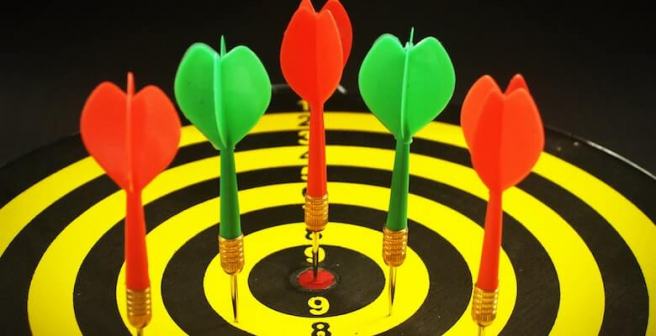
Variance in data measures the extent to which individual data points deviate from the mean or average value. It quantifies the spread or dispersion within a dataset. Understanding variance is crucial because it indicates the stability and consistency of your data.
Heteroscedasticity, the uneven spread of data points, can distort the results of statistical analyses, leading to inaccurate conclusions about relationships between variables. In contrast, homoscedasticity, where data points have consistent variability, ensures more trustworthy and interpretable results.
As a researcher, you need to understand heteroskedasticity and homoskedasticity in order to assess the quality of data, make informed decisions about statistical methods, and interpret the reliability of study findings.
What Is Heteroscedasticity?
Imagine you're studying the effects of a new drug on blood pressure. You collect data from patients, measuring their blood pressure before and after treatment. Heteroscedasticity refers to a situation where the variability in your data points isn't consistent across the range of your independent variable, in this case, the drug dosage.
Why Does Heteroscedasticity Matter?
Heteroscedasticity can lead to problems in statistical analyses. For instance, when running a regression analysis to assess the drug's impact on blood pressure, heteroscedastic data may produce incorrect estimates and wider confidence intervals, making it challenging to draw accurate conclusions.
What Is Homoskedasticity?
Now, picture a scenario where the variability in your blood pressure measurements remains constant across all drug dosages. This is homoskedasticity---the ideal state where the spread of data points is uniform along the entire range of your independent variable.
In our drug study, if the blood pressure measurements show consistent variability, regardless of the drug dosage, you have homoscedastic data.
Why Does Homoskedasticity Matter?
Homoscedastic data makes statistical analyses more reliable. When conducting regression analyses, for example, you can trust the results and make accurate predictions because the variance in your data remains stable.
Detecting Heteroscedasticity
To detect heteroscedasticity, create scatterplots of your data or plot the residuals (the differences between observed and predicted values) against the independent variable. If you notice a funnel-like shape or a pattern where the spread widens or narrows, you likely have heteroscedasticity.
Addressing Heteroscedasticity
You can address heteroscedasticity by using various techniques. Let’s look at the three most popular ones:
Data Transformation: One way to address heteroskedasticity is by transforming your data. Common transformations include taking the logarithm, square root, or inverse of your variables. These transformations can help stabilize the variance, making it more consistent across different levels of the independent variable. However, be cautious when interpreting results after transformation, as they may not directly correspond to the original data.
Weighted Regression: Another approach is to use weighted regression techniques. Here, you assign different weights to each data point based on the level of variance associated with it. Data points with higher variance receive lower weights, and those with lower variance receive higher weights. This gives more importance to observations with less variability, effectively mitigating the impact of heteroskedasticity on your regression analysis.
Robust Standard Errors: When you suspect heteroskedasticity but are hesitant to transform your data or use weighted regression, robust standard errors can be a helpful alternative. Robust standard errors adjust the traditional standard errors in regression models to account for heteroskedasticity. This method provides more accurate confidence intervals and hypothesis testing, even when heteroskedasticity is present, allowing you to make valid statistical inferences.
The choice of method depends on your specific research context.
Conclusion
Understanding heteroscedasticity and homoskedasticity is crucial for biomedical researchers. By addressing heteroskedastic data appropriately, you can enhance the accuracy and reliability of your research findings. So, don’t fear variance in your dataset; there are multiple ways you can address heteroskedasticity and generate robust research results!
Get advice from an experienced biostatistician on how to deal with variability in your research data. Check out Editage’s Statistical Analysis & Review Services.
Comments
You're looking to give wings to your academic career and publication journey. We like that!
Why don't we give you complete access! Create a free account and get unlimited access to all resources & a vibrant researcher community.
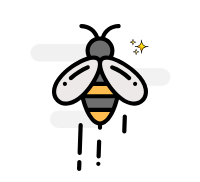
Subscribe to Conducting Research