Choosing the right regression method: A handy guide for biomedical researchers
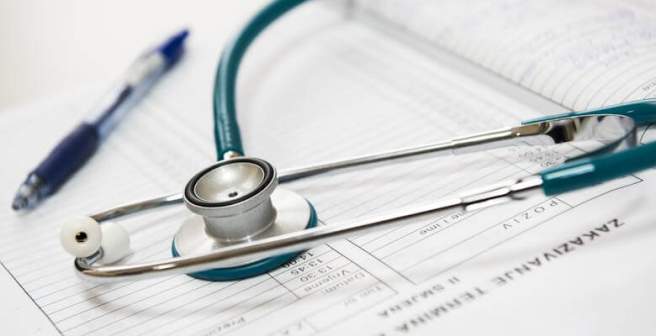
Regression analysis is crucial in biomedical research for understanding relationships between variables, predicting outcomes, and identifying risk factors or treatment effects. It aids in uncovering patterns in complex biological systems. However, researchers often end up with inappropriate regression models, because of overfitting models to noise, not validating assumptions, ignoring multicollinearity, extrapolating beyond the data's scope, etc. Failure to account for confounding variables and inadequate sample sizes can also compromise your results. Choosing an appropriate regression method is essential to ensure the reliability and generalizability of regression data.
Choosing the best regression method depends on several factors, including the nature of your data, the relationship between variables, and the assumptions of the regression models. Here are some guidelines to help you choose the most appropriate regression method:
The Kind of Relationship You are Exploring:
If the relationship between the independent and dependent variables appears to be linear, consider linear regression. If the relationship is not linear, you might need a non-linear regression model, such as polynomial regression, exponential regression, or logarithmic regression.
Number of Variables:
If you have only one independent variable, use simple linear regression. For multiple independent variables, use multivariate regression.
Assumptions:
Linear regression assumes that there is a linear relationship, homoscedasticity (constant variance of errors), independence of errors, and normal distribution of errors. If these assumptions are violated, consider alternative methods.
Data Distribution:
If your data doesn't meet the assumption of normally distributed residuals, you can consider alternative regression methods such as robust regression or quantile regression, or you can try transforming the data (e.g., using logarithmic or Box-Cox transformations). These methods provide more robust parameter estimates in the presence of non-normally distributed residuals.
Outliers:
If your data contains several outliers, consider using robust regression methods, such as robust linear regression or weighted least squares regression.
Collinearity:
If there is high multicollinearity among independent variables (i.e., they are strongly correlated with each other), consider methods like ridge regression or principal component regression.
Sample Size:
If you have a small sample size or limited data, be cautious about overfitting. Regularization techniques like Ridge or Lasso regression can be helpful.
Purpose of Analysis: Prediction vs. Inference:
To predict outcomes with less concern about the underlying relationships, machine learning methods like decision trees, random forests, or support vector regression may be suitable. In contrast, if your goal is to understand the relationships between variables, traditional linear regression or generalized linear models may be more appropriate.
Always keep in mind that the choice of a regression method may involve some trial and error, and it's important to validate the chosen model on independent datasets or through cross-validation to ensure its generalizability. Choosing among the many different regression methods is challenging, but it’s important to carefully consider the merits and use cases for different methods, in order to generate high-quality and trustworthy data.
Unsure of which regression method best suits your data and research question? Consult an expert biostatistician under Editage’s Statistical Analysis & Review Services.
Comments
You're looking to give wings to your academic career and publication journey. We like that!
Why don't we give you complete access! Create a free account and get unlimited access to all resources & a vibrant researcher community.
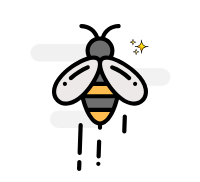
Subscribe to Conducting Research