Survival Analysis and Big Data: A Handy Guide for Biomedical Researchers
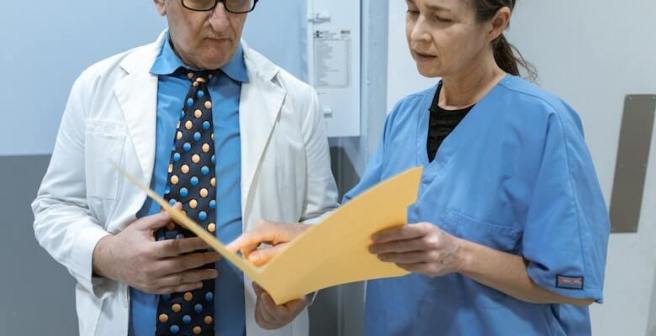
Survival analysis might sound a bit intense, but it’s really just a way to figure out how long things last – whether it’s people’s lives, machines, or even chocolate bars! In the biomedical world, it helps us study how long it takes for something to happen, like a disease appearing or a patient recovering.
One of the strongest factors shaping biomedical research today is big data. We’re talking about loads and loads of information – more than you can imagine. It’s like a giant puzzle made up of patient records, test results, and vast datasets of genomic information. Big data is like a treasure chest, but you need the right tools to unlock its secrets.
Survival analysis using big data is crucial in biomedical and clinical research for several reasons. First, it lets scientists dive into massive datasets with tons of info about patients, helping them spot even the tiniest things that affect survival rates. This can lead to finding brand new ways to predict outcomes and develop better treatments. Plus, it’s like having a magnifying glass on disease progression, which can help catch and treat illnesses earlier. And with big data, researchers are better equipped to spot rare events and variations, making predictions more accurate and healthcare better for everyone.
In this quick and easy guide, we’re going to unravel the mysteries of survival analysis and how it teams up with big data to give us fascinating insights into health and medicine. Let’s get started!
Traditional Survival Analysis Techniques
First, we’ll take a look at some popular statistical tests that have been used for survival analysis.
-
Kaplan-Meier Curve: The Kaplan-Meier curve shows you the probability of survival over time for the members of a group. This curve helps you see the big picture and spot trends that might be hiding in the data jungle.
-
Cox Proportional-Hazards Model: The Cox Proportional-Hazards model is a sophisticated technique that takes factors like age, gender, and treatments into account and tells you how these factors impact survival rates. It doesn’t just show you the survival odds; it helps you understand how different factors work together to shape the outcomes.
-
Log-Rank Test: The log-rank test is a statistical test used in survival analysis to compare the survival distributions of two or more groups or cohorts. It helps determine whether there are significant differences in survival times between these groups. The log-rank test is a non-parametric test, which means it makes no assumptions about the underlying distribution of survival times. It is widely used in survival analysis because it’s relatively easy to understand and implement.
The above tests may not be suitable for big data due to their limitations in handling the sheer volume, complexity, and high dimensionality of data, which can lead to computational inefficiency and make the results hard to understand. For example, when there are tons of data points, your Kaplan-Meier curve might start to look like a squiggly mess. Also, if your data cover a very long time period, the curve might become too detailed and difficult to understand quickly.
New Approaches for Big Data
As mentioned earlier, traditional statistical techniques may not be suitable for survival analysis of big data because they may struggle to handle the high-dimensional, complex, and non-linear relationships present in large datasets. Here’s where new methods come in handy:
Divide-and-combine method
Wang et al. (2022) proposed a technique that breaks a large dataset into smaller subsets and then combines the results in a smart way, using a weighted method. They tested out this approach using +20 years of data from ~73,000,000 emergency room admissions in the US, and were able to identify risk factors related to multivariate cardiovascular-related health outcomes.
Machine learning
Machine learning is already revolutionizing the way we analyze biomedical data, and it’s also got a lot of promise for survival analysis. As shown by Wang et al. (2019), machine learning even outperforms traditional analysis methods, especially when dealing with censored data (i.e., data where the event of interest has not occurred for one or more subjects during the study period). To delve further into this topic, you could take a look at Spooner et al. (2020)‘s useful comparison of the performance and stability of ten machine learning algorithms, combined with eight feature selection methods, for survival analysis of high-dimensional, heterogeneous, clinical data.
Deep learning
Like machine learning, deep learning also looks very promising as a tool for conducting survival analysis. Deep learning is useful for survival analysis due to its ability to automatically learn complex patterns from diverse data sources, improving the prediction of time-to-event outcomes. Wiegrebe et al. (2023) have published a useful preprint on currently available deep learning-based methods for survival analysis, reviewing their theoretical dimensions as well.
Conclusion
In conclusion, using big data for survival analysis helps us uncover hidden patterns, discover new ways to predict outcomes, and make treatments as unique as each patient. With the power of massive datasets, we can peer deeper into disease progression, catching it early and improving patient care.
Ready to harness the power of big data when conducting survival analysis? Team up with an expert biostatistician, under Editage’s Statistical Analysis & Review Services.
Comments
You're looking to give wings to your academic career and publication journey. We like that!
Why don't we give you complete access! Create a free account and get unlimited access to all resources & a vibrant researcher community.
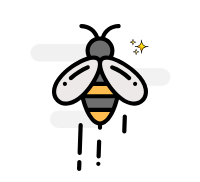
Subscribe to Conducting Research