What biomedical researchers need to know about T tests
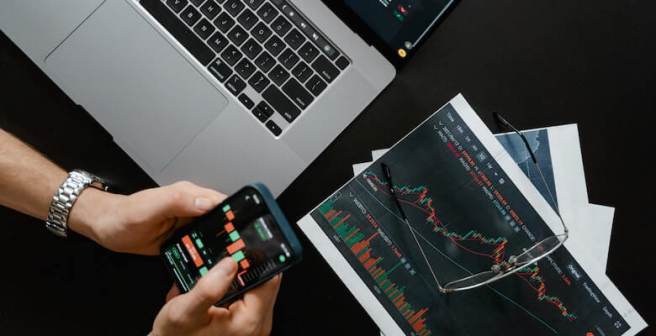
If you're venturing into the world of statistics and data analysis, the t-test is likely to become your trusty companion. This simple yet powerful tool helps you compare the means of two groups, making it a fundamental test in biomedical research. But before you run any analyses, let's cover some key points you need to know to ensure accurate and meaningful results.
Understanding the T-Test
Let's start with the basics. The t-test is used to determine if there is a significant difference between the means of two groups. It assesses whether the observed difference is genuine or just due to random chance. Understanding the t-test's purpose is crucial to interpreting your results correctly.
Types of T-Tests
There are two main types of t-tests: the independent samples t-test and the paired samples t-test. The independent samples t-test compares two unrelated groups (e.g., participants with and without a history of cancer), while the paired samples t-test analyzes related groups, like pre and post-treatment measurements. Selecting the appropriate type of t-test is essential for the accuracy of your conclusions.
Assumptions of the T-Test
Like any statistical test, the t-test has certain assumptions that must be met for reliable results. These include:
Normality: The data in each group should follow a normal distribution.
Independence: For the unpaired t test, observations in one group should not influence the other group’s observations.
Homogeneity of Variance: The variance within each group should be roughly equal.
Don't panic if your data violates some of these assumptions. You can use robust statistical methods or transformations to address these issues.
Sample Size Matters
The t-test's reliability is heavily influenced by the sample size of your groups. Larger sample sizes provide more robust results, whereas small samples can lead to unstable outcomes. Aim for sufficient sample sizes to ensure the statistical power necessary to detect meaningful differences.
Effect Size: Actual Differences
Looking beyond statistical significance, consider the effect size and statistical power of your study. Effect size quantifies the magnitude of the difference between groups, while power estimates the likelihood of detecting an effect if it exists. Both are essential for meaningful interpretations and designing well-powered studies.
For example, when comparing two interventions A and B to lower blood pressure, you might find a significant difference in mean blood pressure between the A and B groups post-intervention. But if the mean difference is just 2.2 mmHg (which is not clinically meaningful), you can’t say that A is necessarily more effective than B.
When reporting your t test results, it’s important to provide the means and standard deviations of each pair of groups being tested, so that readers can gauge effect sizes.
Interpreting P-Values
The p-value is a common metric in hypothesis testing. It indicates the probability of obtaining results as extreme as those observed if the null hypothesis (no difference) were true. However, remember that a small p-value in your t test doesn't necessarily mean the difference detected is meaningful or valuable in real life. Always combine p-values with effect size and contextual knowledge.
Conclusion
The t-test is a valuable tool for biomedical researchers, but its proper use and interpretation are crucial. By understanding its purpose, assumptions, and effect size, you'll be well-equipped to run meaningful and reliable analyses. Remember, statistical tests are just one part of the research process, so always interpret your results in the broader context of your study.
Need help in assessing the differences between groups in your study? Our expert biostatisticians can help you choose, run, and interpret the results of the right test. Check out Editage’s Statistical Analysis & Review Services.
Comments
You're looking to give wings to your academic career and publication journey. We like that!
Why don't we give you complete access! Create a free account and get unlimited access to all resources & a vibrant researcher community.
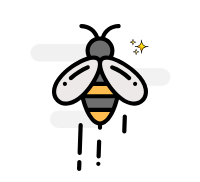
Subscribe to Conducting Research