Efficient, cost-effective, and patient-centered: How machine learning can transform the way we conduct clinical trials
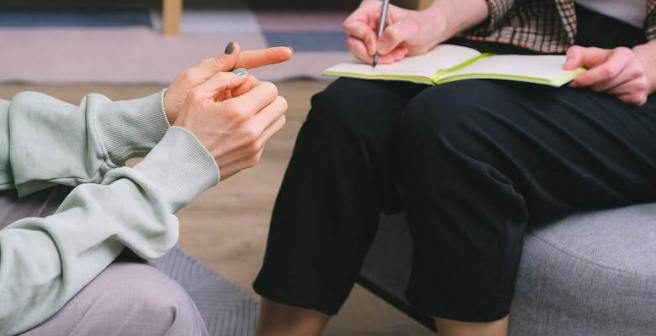
Machine learning is transforming how clinical trials are conducted. This groundbreaking synergy of technology and medicine offers more efficient, cost-effective, and patient-centered trials. In this overview, we'll examine how machine learning benefits various aspects of clinical trial management, from planning the study to overseeing participants and handling data.
Trial Protocol Optimization
Machine learning can aid in designing more efficient clinical trial protocols by analyzing historical data from similar trials. It can optimize variables such as sample size, duration, and endpoints, enhancing the trial's feasibility and cost-effectiveness. See, for example, the machine learning pipeline developed by Kavalci and Hartshort (2023) to reduce the risk of wasted resources in a clinical trial and thus optimize trial design.
Clinical Trial Participant Management
a. Selection of Patient Populations for Investigation:
Machine learning can identify patient characteristics and biomarkers that are most likely to respond to the investigational treatment, helping to select appropriate study populations. Al-Tashi et al. (2023) provide an in-depth review of how machine learning can be used to identify prognostic and predictive cancer biomarkers.
b. Participant Identification and Recruitment:
Machine learning can analyze electronic health records and other data sources to identify potential participants, streamlining the recruitment process and ensuring a more diverse participant pool. Miller et al. (2023) have explained in detail how machine learning facilitates participant identification in neurology research.
c. Participant Retention, Monitoring, and Protocol Adherence:
Machine learning can predict which participants are at risk of dropping out or not adhering to the protocol. This enables early intervention to improve participant retention and data quality. Hope Weissler et al. (2021) discuss in detail ways in which machine learning can reduce the burden on trial participants, right from using facial recognition technology to monitor protocol adherence to using natural language processing to identify adverse events in social media posts by patients.
Data Collection and Management
a. Collection, Processing, and Management of Data from Wearable and Smart Devices:
Machine learning can process and analyze data from wearables and other smart devices in real-time, providing continuous monitoring and reducing the need for frequent in-person visits. Abiodun et al. (2022) developed a conceptual framework for using machine learning for remote monitoring in a clinical trial.
b. Approaches to Missing Data:
Machine learning can impute missing data by leveraging patterns and correlations within the existing dataset, ensuring that valuable information is not lost. See, for instance, how Haliduola et al. (2022) used machine learning strategies to impute missing information in clinical trials.
c. Data Analysis:
ML techniques like predictive modeling and feature selection can help identify meaningful insights from the collected data, improving the quality of clinical trial outcomes. For example, Kalscheur et al. (2018) used machine learning to predict patient outcomes following cardiac resynchronization therapy, using data from the data from the Comparison of Medical Therapy, Pacing and Defibrillation in Heart Failure (COMPANION) trial.
Barriers to Using Machine Learning in Clinical Trials
a. Data Privacy and Security:
Protecting patient data is crucial, and regulatory compliance like HIPAA and GDPR can complicate data sharing and analysis.
b. Data Quality and Standardization:
Ensuring the quality and standardization of diverse data sources is challenging but essential for meaningful ML analysis.
c. Regulatory Approval and Validation:
ML models need validation and regulatory approval, which can be time-consuming and costly.
d. Interoperability and Integration:
Integrating ML systems with existing clinical trial workflows and EHRs can be complex.
e. Ethical and Bias Concerns:
ML algorithms can inadvertently perpetuate biases present in historical data, necessitating careful model development and monitoring.
f. Cost and Resource Constraints:
Implementing machine learning may require additional resources and expertise, which can be a barrier for some research organizations.
Conclusion
Incorporating machine learning into clinical trials offers enormous potential to optimize protocols, enhance participant management, and improve data collection and analysis. However, addressing the associated barriers is crucial for successful adoption and the realization of its benefits in biomedical research.
Ready to leverage machine learning to make your clinical trial more efficient? Consult an experienced biostatistician under Editage’s Statistical Analysis & Review Services.
Comments
You're looking to give wings to your academic career and publication journey. We like that!
Why don't we give you complete access! Create a free account and get unlimited access to all resources & a vibrant researcher community.
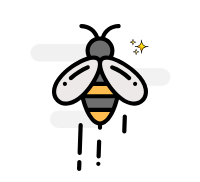
Subscribe to Conducting Research