Exploring the magic of Markov Chain Monte Carlo: A biomedical researcher’s guide
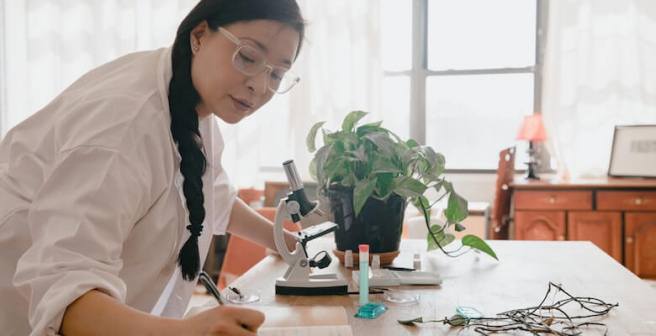
Imagine you have a complex Bayesian model, like predicting the effectiveness of a new drug. The traditional methods may not work very well because they rely on mathematical shortcuts or simplifications. This is where Markov Chain Monte Carlo (MCMC) methods shine. These methods allow us to handle these complex models and explore the full range of possibilities. In this blogpost, we’ll explore the magic of MCMC and how to leverage its potential in biomedical research, starting with a quick explanation of what MCMC actually is.
Markov Chains
Think of a Markov chain as a series of connected events where the outcome of each event depends only on the previous one. In our biomedical context, this could be the progression of a disease in a patient over time. Each step only depends on the previous state.
Monte Carlo Simulation
Monte Carlo is like playing with randomness to find answers. Imagine we want to know the average age of patients in a clinical trial. Instead of surveying all of them, we can randomly select a few and estimate the average. Repeat this many times, and we get a pretty good estimate.
Putting It Together: Markov Chain Monte Carlo (MCMC)
MCMC combines Markov chains and Monte Carlo simulations. It’s like playing with randomness in a smart way. Here’s how it works:
Start Somewhere: We begin with an initial guess, or starting point, in our problem. For our drug example, this could be an estimate of its effectiveness.
Random Walks: We take small, random steps from our starting point. These steps mimic uncertainty or noise in our data.
Accept or Reject: We decide whether to keep these new guesses based on how well they fit our data. The better they fit, the more likely we are to accept them.
Repeat: We repeat this process many, many times. Over time, our guesses start to converge towards a meaningful result.
Convergence in MCMC
Convergence refers to the state where the sampled values in the chain stabilize and reach a stationary distribution. It signifies that the MCMC algorithm has explored the probability distribution thoroughly, and further iterations don’t substantially alter the results. In biomedical research, this means that the MCMC process has effectively estimated model parameters or the posterior distribution (i.e., the updated probability distribution of variables after we incorporate the observed data). Convergence is important because it allows researchers to make reliable inferences and conclusions from the data.
Tuning Parameters in MCMC
Tuning parameters refer to the settings that control how the MCMC algorithm explores and samples from the probability distribution of interest. These parameters include the step size or proposal distribution, which dictate the size and direction of the random jumps taken in the parameter space during each iteration of the chain. Properly tuning these parameters is essential because they influence the efficiency and effectiveness of the MCMC process. If the tuning parameters are set poorly, the MCMC chain may converge slowly, get stuck, or fail to adequately explore the target distribution, and your results become unreliable. It’s crucial to find the right balance for these parameters when you’re using MCMC for complex modeling tasks.
Advantages of MCMC:
Flexibility in Complex Models: MCMC shines when dealing with complex models. In biomedical research, this flexibility is vital. For instance, when modeling the progression of a disease in a patient, the relationships between variables can be intricate and difficult to capture with simpler methods.
Uncertainty Quantification: MCMC provides a way to quantify uncertainty. In drug development, researchers need to estimate the effectiveness of a new treatment. MCMC allows them to not only estimate the effectiveness but also quantify how certain or uncertain they are about that estimate.
Handling Missing Data: Biomedical data can often be incomplete. MCMC can handle missing data elegantly by imputing missing values based on the observed data. For example, when analyzing patient records, MCMC can estimate missing lab results.
Sampling from Complex Distributions: Sometimes, researchers need to sample from very complex probability distributions. In genomics, when estimating the distribution of gene expression levels, MCMC can efficiently handle these high-dimensional and intricate distributions.
Disadvantages of MCMC
Computational Intensity: MCMC can be computationally demanding, especially for large datasets or highly complex models. For instance, when studying the genetic basis of diseases, MCMC may require significant computational resources.
Difficulty in Tuning Parameters: Properly tuning MCMC algorithms can be challenging. Selecting the right step size or proposal distribution is crucial. Choosing the wrong tuning parameters can lead to inefficient sampling and inaccurate results.
Challenges in Reaching Convergence: MCMC chains must converge to the true distribution; otherwise, the results are meaningless. For instance, in pharmacokinetics, if the chains don’t converge well, the estimated drug concentration profiles may be unreliable.
Necessity for Appropriate Initial Values: The choice of initial values can affect the results. For example, in cancer research, starting MCMC with inappropriate initial estimates of tumor growth rates can lead to inaccurate predictions.
Popular MCMC Algorithms
Let’s take a look at the most commonly used MCMC algorithms in biomedical research:
Metropolis-Hastings (MH): MH is like trying different medicines for a patient’s illness. We make guesses, see if they work better or worse, and decide whether to stick with them. For example, when figuring out the right drug dosage for a patient with a chronic condition, we adjust it based on their response, improving treatment.
Gibbs Sampling: Think of Gibbs Sampling as solving a jigsaw puzzle piece by piece. If you have a patient’s medical history and want to predict future health, you focus on one aspect at a time, like diet, exercise, or genetics, and then combine them to get the full picture.
Hamiltonian Monte Carlo (HMC): HMC is like navigating a maze efficiently. When researching genetic mutations linked to diseases, HMC helps explore all possible routes through the maze (genetic variations) quickly, finding the important ones faster.
Slice Sampling: Slice Sampling is like making a sandwich with layers of different ingredients. In studying the interactions between multiple drugs in cancer treatment, Slice Sampling helps us find the best combinations without guessing ingredient proportions – it takes a slice at a time until we get the perfect recipe.
Conclusion
MCMC might sound intimidating, but it is a powerful tool when you’re dealing with complex data and challenging research questions. Its ability to navigate complex models, quantify uncertainties, and adapt to evolving data sets it apart as a trusted ally in your research journey. As you venture further into the realms of personalized medicine, disease modeling, and drug development, remember that MCMC offers the power to turn uncertainty into insight and complexity into clarity.
You can now confidently leverage the power of MCMC and other complex methods in your biomedical research project! Consult an expert biostatistician under Editage’s Statistical Analysis & Review Services.
Comments
You're looking to give wings to your academic career and publication journey. We like that!
Why don't we give you complete access! Create a free account and get unlimited access to all resources & a vibrant researcher community.
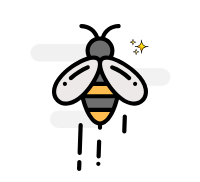
Subscribe to Conducting Research