Is my research significant? Why you shouldn't rely on p values
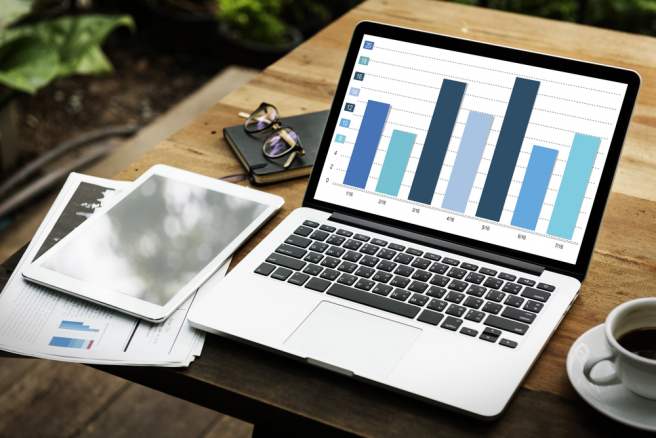
The most commonly reported statistic in research papers may also be the most misunderstood and misused. We’re talking about the p value.
Recently, the American Statistical Association (ASA) released the “Statement on Statistical Significance and P-Values,” outlining six principles pertaining to appropriate use and interpretation of p values. The full statement is available here.
Let’s take a look at the ASA principles, and how they apply to research.
1. P values can indicate how incompatible the data are with a specified statistical model.
Here, the important word is “specified.” Remember that in any study or analysis, the researchers are bound to have made certain assumptions when creating a statistical model. According to statisticians, a p value of 0.05 does not mean that there is a 95% chance that a given hypothesis is correct. Instead, this value means that if the null hypothesis is true and if all other assumptions made are valid, we have a 5% chance of obtaining a result at least as large as the result currently obtained.
2. P values do not measure the probability that the studied hypothesis is true, or the probability that the data were produced by random chance alone.
Researchers often erroneously interpret smaller p values to mean that the null hypothesis is false. Actually, p values only indicate the probability of obtaining results at least as large as those observed if the null hypothesis was true.
3. Scientific conclusions and business or policy decisions should not be based only on whether a p-value passes a specific threshold.
“P < .05” is not a guarantee that something is true. Ultimately, a p value is just a statistic and not a sign from heaven. A p value can be influenced by many aspects of a study, especially sample size. If a sample is particularly large, the p value may almost always be significant (though the effect size may be negligible), unless there is absolutely no effect. Hence, it’s common sense that you can’t make a practical decision on the basis of a p value alone.
4. Proper inference requires full reporting and transparency.
Often, the only results reported are those with p value below .05. The ASA strongly discourages this kind of “cherry picking.” Instead, it recommends that authors report all hypotheses explored, all statistical analyses conducted, and all p-values obtained, whether significant or not. Only then can authors draw valid conclusions on the basis of their data. Authors can take the help of professional publication support services, for example, Editage’s Statistical Review Service, to ensure robust analyses and and get their statistical analyses reviewed.
5. A p-value, or statistical significance, does not measure the size of an effect or the importance of a result.
Some authors label findings with a very low p value (<.001) as “very significant” or “highly significant.” However, a low p value does not mean that the result is of practical or clinical importance.
Let’s assume you’ve found a statistically significant relationship between increased energy drink consumption and positive body image in girls. This doesn’t mean that you should design an intervention to improve body image in which girls are given free energy drinks! Instead, you should look at the strength of the relationship (e.g., correlation coefficient, regression coefficient). If the relationship is weak (e.g., a correlation coefficient of just 0.1), your intervention will probably be more effective if you consider other factors that have a stronger relationship with body image (e.g., general self-esteem, frequency of fat talk).
It’s also important to consider context when determining the importance of a result. Small differences between large groups can be statistically significant but unimportant practically, while large differences between small groups can be important in practical terms even if they are not statistically significant. For example, a mean increase of 1.5 points in scores on a 100-point math test after an educational intervention may be statistically significant, but the intervention itself may not be particularly beneficial or useful in real life.
6. By itself, a p-value does not provide a good measure of evidence regarding a model or hypothesis.
Authors should avoid reporting just p values in their results. A small p value doesn’t indicate that the null hypothesis is false, nor does a large p value mean that the null hypothesis is true. In research, there could be a variety of hypotheses that are just as consistent with the observed data. Hence, a p value is not the only form of statistical support for the model or theory being tested, and the value of a study does not depend solely on the p values found.
In summary, although p values can be useful, they are not the yardstick by which a study becomes valuable and important, and they should not be treated as such. Statistical significance is not the same as scientific, practical, or clinical significance.
Related reading:
Linking statistical significance to clinical importance of trial data: A paradigm shift
Published on: May 11, 2016
Comments
You're looking to give wings to your academic career and publication journey. We like that!
Why don't we give you complete access! Create a free account and get unlimited access to all resources & a vibrant researcher community.
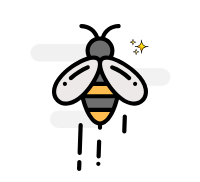